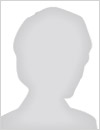
This will count as one of your downloads.
You will have access to both the presentation and article (if available).
This will count as one of your downloads.
You will have access to both the presentation and article (if available).
Hyperspectral imaging is an emerging technique in remote sensing. The very high spectral resolution in the hundreds of acquired images provides the potential of more accurate detection, classification, and quantification than that obtained using traditional broad-band multispectral imaging sensors. However, the resulting high data dimensionality poses challenges in data analysis. This course explains basic principles of dimensionality reduction that can maintain or even improve the performance of hyperspectral data analysis tasks (e.g., detection, classification).
The primary goal of this course is to introduce the preferred feature extraction and feature selection algorithms for hyperspectral imagery. Specifically, two types of dimensionality reduction techniques, transformation-based and band-selection-based, will be studied. For each category, both supervised (with known class types and samples) and unsupervised (without any prior knowledge) approaches will be presented. In addition to the widely-used approaches, the state-of-the-art algorithms (e.g., manifold learning, sparse regression) will be discussed. Performance of all the techniques is evaluated using several real data experiments.
View contact details
No SPIE Account? Create one