1.IntroductionPulmonary embolism (PE) refers to a clinical and pathophysiological syndrome that commonly occurs as a cardiovascular disease.1 It shows pulmonary circulation disorders and even pulmonary infarction that are caused by endogenous or exogenous emboli block of the pulmonary artery or its branches. According to a report,2 the incidence of PE was the second only to coronary heart disease and hypertension in cardiovascular diseases, which is equivalent to 1/2 of the incidence of acute myocardial infarction; the mortality rate ranks third, the clinically observed acute PE mortality rate is not lower than that of myocardial infarction.3 The most accurate PE detection method is the pulmonary artery digital subtraction angiography (DSA), which is the gold standard for the PE diagnosis with almost 100% accuracy. However, this examination method is invasive and easy to cause damage to blood vessels or surrounding tissues. Besides, complications frequently occur after examination that also limit the DSA application.4 Another frequently used clinical noninvasive detection is the computer tomography pulmonary angiography (CTPA), which can detect pulmonary artery emboli through morphological character analysis, thus providing a basis for the PE diagnosis. However, due to the complex vascular structure below the pulmonary artery subsegment and the large interference outside the slice, the diagnostic efficiency of this part of embolus is reduced. Therefore, noninvasive detection of PE remains a challenge in cardiovascular medicine. Near-infrared spectroscopy (NIRS) is based on the spectral characteristics of oxygenated hemoglobin (HbO2) and deoxygenated hemoglobin (HbO)5 and quantitative determination of tissue hemoglobin oxygen saturation for measure disease. Since its invention in the 1970s, with its advantages of noncontact, noninvasive, and online rapid detection, it has been used in a series of clinical human body studies with validated results.6 In recent years, great progresses in NIRS research were on human brain,7,8 heart,9 lung,10,11 and mammary gland.12 Accurate tissue modeling of tissue increases the utility of photon migration for a broader range of applications. NIRS can detect up to a few centimeters that are enough to penetrate the thorax and reach the pulmonary artery.13 However, the study of photon migration in human pulmonary artery region has not been thoroughly studied, and noninvasive optical technology has great prospects in clinical PE detection. In previous studies, hemodynamic abnormalities occurred first with PE, followed by characteristic events associated with hypoxemia. It was evident that the right ventricular blood reperfusion into the pulmonary artery was in disorder under the instance of pulmonary artery lesions (e.g., increased levels of embolism). The changes of hemodynamic provided physiological basis for direct detection of PE under hypoxemia.14 At present, the migration rules of NIR light in the pulmonary artery are not clear, so it is impossible to determine where to place the light source and detector (LSD) and how much the separation of the LSD can better detect the signal sensitivity distribution (SSD). This is also an urgent problem to be solved to achieve the clinical application of noninvasive PE detection. It is very important to obtain the quantitative characteristics of photon migration in the thoracic tissues model under noninvasive detection mode and to study the optimal design of light source detector placement. This study aimed to solve these problems. Before to know whether PE could be detected by the optical method, the photon transmission rules in pulmonary tissues were needed to understand. The photon transmission of human body was complicated. To be more clarified, the pulmonary artery study was simulated on a three-dimensional (3D) structure, VCH is the best choice. The VCH is an individualized 3D heterostructure model,15 which is suitable for the simulation of 3D voxel media. The VCH has played an important role in many studies.16–18 The Monte Carlo code MCML (Monte Carlo modeling of light transport in multilayered tissue) has been the gold standard for simulations of light transport in multilayer tissue,15,19 but it is ineffective in the presence of 3D heterogeneity. In fact, the team has developed a software program targeted for Monte Carlo simulation of light propagation in voxelized media (MCVM).16 Using the MCVM, we can better evaluate the relationship between photon propagation in VCH chest model and the layout between LSDs. At present, MCVM + VCH has been successfully used for studying the photon transmission distribution of brain, mammary gland, and heart, which showed the reliability of the MCVM+VCH method. However, this method has not been used for obtaining the photon migration characteristics of pulmonary artery yet. In this study, thoracic tissues model of VCH dataset was adopted to improve the precision of chest structure modeling, and Monte Carlo simulation was carried out in combination with 3D voxelized media to establish a quantified and visualized photon migration human chest model. The SSD, the partial path-length factor (PPF),15 the differential path-length factor (DPF),16 and pulmonary artery signal contribution ratios of a series of separation of the LSD were calculated. The results showed that the degree of PE had a strong linear relationship with the detected signal intensity. We simulate and visualize photon migration in human thoracic tissues with MCVM + VCH and the potential of noninvasive PE detection was first studied. 2.Materials and Methods2.1.Thoracic Model Built from the VCHThe simulation was operated on a thoracic model established from the VCH dataset, which was a set of color photographs from the whole-body frozen sections. The VCH dataset was collected from a standing frozen Chinese male body (a donated cadaver) with little pathological alternations. In this study, we used 80 slices of photographic images of the VCH sections to complete the thoracic tissues region of the pulmonary artery from top to bottom, a photographic thoracic model of was first built (Fig. 1). One voxel was defined as a cubic. The whole model was divided into eight parts according to tissues that included skin, muscle, bone, subcutaneous fat, lung, Pa vessel wall, arteries, and venous blood. Please note that the voxels outside the thoracic tissues were assigned as air and did not participate in Monte Carlo simulation. Fig. 1The description of VCH thoracic models. (a) A representative original thoracic photography image from VCH. (b) The thoracic model was divided into eight tissue parts. (c) A representative graph of the pulmonary artery structure (an open access picture from 3D Human Body app). (d) The thoracic model was built to the 3D matrix of . ![]() 2.2.Monte Carlo SimulationMonte Carlo simulation was used to deduce light propagation in the VCH of thoracic tissue. The Monte Carlo software used here was targeted for 3D voxelized media, the algorithm of which has been described in previous literature.20 In theory, when a photon packet (or a single photon) entered a tissue, it would be randomly scattered or continuously absorbed by the tissue media until it died.21 Survival photons collected by the detector could be analyzed to get the valued information, such as intensity and propagation path length. In this study, a light source was placed upon in between the second and third anterior ribs, the detector was attached to the skin on the horizontal left side of the light source, and the axis directions were shown in the Fig. 2. The input light was the 800-nm IR light and other set up parameters were the same with the previous studies and shown in the Table 1.30–32 Each simulation ejected . After 10 times simulation, the average photon fluence was calculated for representing the light propagation results. Besides, two files were generated for respectively recording the absorbed photon numbers and output photon distribution. According to these two files, a series of photon transmission characters were calculated. Fig. 2Light fluence distribution in thoracic tissue under field of view. (a) The position of the LSD on the human thoracic model. (b) The three-dimensional space coordinate system used in this study. (c) Light fluence in the plane scale is . (d) Light fluence in the plane scale is . (e) Light fluence in the plane scale is . ![]() Table 1Optical properties of thoracic tissue at 800 nm.
Where n is the refractive index of the medium, μa is the absorption coefficient, μs is the scattering coefficient, and g is the anisotropy factor. Three disease levels were designed for simulating the optical transmission on the patients. We change the relationship between arterial blood and venous blood parameter to change the blood oxygen saturation.33 According to the variation of the arterial oxygen blood saturation () and the venous blood oxygen saturation (), four groups were used in this study, including the three patient groups with mild (Level I), medium (Level II), and serious (Level III) disease, and the control group of normal people.34 The parameter details were shown in the Table 2. Table 2The parameters of SA and SV for the four groups.
SaO2 was the arterial blood oxygen saturation.SvO2 was the venous blood oxygen saturation. 2.3.Spatial Sensitivity DistributionOne output file of MCVM program contained the photon absorption in every voxel of the whole tissue model. The spatial distribution of light absorption (),35 a 3D matrix of , can be obtained from this file. Equation (1) was used to calculate the weight of the light fluence () from the light source coordinate position () to a random voxel () position ().35 The was the absorption coefficient of the tissue type at the ’th voxel.35 Then () of the individual voxel was calculated by the three-point Green’s Eq. (2).36 indicated the light fluence of a voxel () at the () location.36 After collecting all the SSD value of voxels, the SSD of the whole model was calculated by summing all these voxel SSD value.36 At the same time, the SSD of a specific tissue was calculated by summing all the voxel SSD value of this tissue.37 The ratio of the SSD of a specific tissue to total SSD represented the involvement of this tissue type in the light transportation35–37 2.4.Separation of the LSDThe most optimal separation of LSD was calculated. As mentioned above, the light source was placed between the second and third anterior ribs, where had few muscles and could penetrate more photons. The detector was placed horizontally to the left of the light source. The separation of the LSD was determined by two factors, such as DPF and PPF. DPF was the scaling factor that relates source-detector separations to the average path length that light travels. The notion of a DPF implies the assumption that the chromophore change was global to the sampling region.38 In contrast, PPF refers to a similar scaling factor but one that assumes a focal change. A PPF therefore represents a proportionality factor converting the source-detector separation into the average path length that light travels through a focal region of chromophore change.39,40 The PPF of the pulmonary artery (PPFpa) was calculated from the file that recorded the output photon distribution. It represented the sensitivity of the detected light intensity signal to the pulmonary artery.26 The PPFpa/DPF represented the contribution of pulmonary arterial tissue to the absolute absorption of photons. The optimal separation distance between the LSDs was reflected by such indicators of PPF and PPFpa/DPF. 3.Results3.1.Light Fluence DistributionAs mentioned in the method part, light fluence distribution were drawn for indicating the photon existence in the thoracic tissues (Fig. 2). Penetrated photons could be observed reach to the pulmonary artery from any of the three views, such as , , and . From the view, it was proved that the 800-nm light actually traveled through the sternum into the ribcage [Fig. 2(c)]. From the light fluence distribution, it could be observed that photons scattered at the main pulmonary artery [Fig. 2(e)]. The distribution located at 33 mm toward the skin surface proved that photons could penetrate tissues to reach the pulmonary artery [Fig. 2(d)]. A representative light fluence distribution on the 40th slice of view was shown in the [Fig. 3(a)]. It showed that the light intensity was set up to have photons traveling at least 37 mm in the body. The light influence intensity in log10 scale decreased as photons traveled through the tissues from skin, fat, muscle, fat, and pulmonary artery to venous blood [Fig. 3(b)]. Similarly, the light influence intensity decreased sharply in the normal scale.41 By a 3D coordinate system, set a center point position on the body surface (161, 333, and 40) and keep the -axis and -axis unchanged. The photon attenuates from the incident point until the light intensity is close to 0. The distance from the incident point to the end point is the penetration depth.17,42 Fig. 3The light influence intensity changed with tissue depth. (a) The light fluence distribution on the 40th slice. (b) The light fluence intensity decreased from skin to venous blood. ![]() When the depth increased to the pulmonary artery surface, the intensity was 45/voxel. At the depth of 36 mm, the intensity decreased to 1/voxel, at which venous blood was. These data suggest that 800-nm photon could transport into the pulmonary artery at depth of 36 mm with a light source placed upon anterior sternum. Furthermore, the light intensity decreased about times when the photons reached the pulmonary artery vessel wall. 3.2.Effect of Source–Detector Separationaffected spatial sensitivity profiles.43 In this study, different was used for simulating the light propagation in the VCH thoracic lungs. The Fig. 4 showed the SSD on the 40th slice at various . The results showed that the penetration depth and detection area of the spatial sensitivity profile gradually increased when the increased from 1.0 to 3.0 cm. When the increased from 3.0 to 4.0 cm, the detection area of the spatial sensitivity profile become broader but the penetration depth gradually decreased. 3.3.Optimized Source–Detector SeparationThe optimal separation of the LSD was depended on PPFpa, PPFpa/DPF, and pulmonary artery photon absorption. As shown in Fig. 5(a), DPFs tended to increase exponentially with the incremental . Figure 5(b) showed that there was a different performance of PPFpa with . When the was in the range of 2.5 to 2.9 cm, the PPFpa increased rapidly. And then, the PPFpa dropped sharply. Figure 5(c) showed that the change of PPFpa/DPF was affected by . When the was in the range of 2.0 to 3.1 cm, the ratio of PPFpa/DPF increased gradually and then decreased sharply. There was a peak in between the 2.0 and 3.1 cm of . By comparing the Figs. 5(b) and 5(c), both the PPFpa and PPFpa/DPF gradually increased to the maximum value when the increased from 2.0 to 2.8 cm and then sharply dropped when the increased from 2.8 to 3.1 cm. Figure 5(d) showed the absorption of photons by main pulmonary artery at different . The absorption of photons rose slowly at initial and then decreased when the increased from 1 cm. The most optimal was about 2.8 to 3.1 cm where the photon adsorption reached the maximum of 4.6%. These results suggested that the optimized source-detector separation was in between the 2.8 to 3.1 cm. 3.4.Pulmonary Embolism Impact on SSDThe SSD also reflected the grade of PE.44 Table 3 showed the SSD proportion of each tissue at different PE levels. In the pulmonary artery, the SSD proportions were 0.0131%, 0.0129%, 0.0127%, and 0.0126%, respectively, for the healthy people, Level I patient, Level II patient, and Level III patient. When the PE becomes serious, the SSD proportion decreased. The SSD proportions of arterial and venous blood, as well as lung at different PE degrees were also estimated. It showed that the SSD proportion in lung and venous blood slowly decreased when the PE level increased. However, the SSD proportions in arterial blood were almost zero. These results indicated that the venous blood and lungs could be possibly used for detecting the PE by the SSD. Table 3The proportion of SSD (%) of each tissue at different levels of PE.
3.5.Pulmonary Embolism Impact on Detected Light IntensityIn the result of previous part, the optimal source–detector separation was around 2.9 cm. Hence, the was set to 2.9 cm for detecting the light intensity. In the result, light intensities were normalized to find the regular pattern (Fig. 6). Results showed that the normalized light intensity decreased as the PE level increased. Therefore, the light adsorption increased as the PE level increased. Though more photons were absorbed by the higher levels of PE, escaped photons were still detected by the detector. It proved that this detection method could be used for detecting the PE. The linear fitting function of normalized light intensity was (, ). This linear relationship suggested that the PE level could be accurately estimated by the detected light intensity.45 4.Discussion and ConclusionIn this paper, the Monte Carlo simulation based on the VCH was used for simulating the light migration in human thoracic tissues. Importantly, the number of absorbed photons and light fluence distribution in thoracic tissues were indicated partial photons could reach to the pulmonary artery. Besides, the different levels of PE impact on photon detection were confirmed by venous blood SSD and output photon intensity. In addition, the detected light intensity was linearly related to the PE level. These results suggested that PE could be accurately diagnosed by NIRS. In the end, the optimized , 2.8 to 3.1 cm, was calculated by PPF, DPF, and the proportion of absorbed photons. The optical parameters selected in this paper were obtained by the previous work of our group.16,36,46 These parameters were from studies of various groups,22–24,27 which were evaluated and confirmed by previous studies.35,47 In this study, the simulation experiment was carried out in an ideal condition, that was, the influence of the connection between organizations was ignored. Parameters that measured directly from the real human body were not suitable for this study. In the future work, more reality conditions will be included in simulation conditions. Then, the selected parameters will be changed. In Pifferi’s study, optical parameters of lung tissue were measured from the real human body, which included the influence of other tissue reaction.10,11 These parameters will be selected in the future work. The NIR light has better performance in penetration depth.48 The pulmonary artery tissues get more light because of the low absorption coefficient of other tissues in NIRS, as presented in Table 1. In addition, the light source was placed on the skin upon the anterior sternum in this simulation. In the anterior sternum region, there were fewer muscles, and the distance between the skin and the pulmonary artery was relatively small, so the depth of the photon radiation into the pulmonary artery was greater. The photon distribution in the pulmonary artery tissues has been clearly displayed in Fig. 2. Moreover, the results suggest that certain photons may migrate to the surface of the body with optical information of the pulmonary artery tissues. After the incident light enters the human body, photons were absorbed and scattered in different tissues, and partial photons penetrate through the human skin, fat and bone then into the pulmonary artery. The detector we use is a very common OPT101 (OPT101 Monolithic Photodiode and Single-Supply Transimpedance Amplifier).49 The sensitivity of this detector expressed by noise effective power is about (800 nm), the size of photodiode is , and the size of OPT101 is . The OPT101 minimum detectable SSD signal is of order to , so our measured SSD signal of 0.002% is still theoretically within the measurable range of existing devices. During simulation, we can set the receiving area of the detector to make it as consistent as possible with the detection area of the detector in reality. As shown in Table 3, pulmonary artery proportion of SSD was about 0.0131% of the total SSD, Venous blood only accounts for 0.002%. These values indicate that the photodetector can capture those photons that carry optical information about the target tissue and can analyze the captured signal. Our results identified the optimized separation of the LSD depending on the DPF and PPF in Fig. 5. The largest diameter in the thoracic model can be narrowed down to 2.8 to 3.1 cm. In previous study, the optimal source-detector separation was narrowed down to 3 to 3.5 cm in the head model.50 PPFpa/DPF represents the contribution of the pulmonary artery to the absolute absorption measurement, and higher proportion value might be conducive to detection of absolute concentrations of oxyhemoglobin and deoxyhemoglobin to account for hemodynamics at the pulmonary artery. Actually, the separation distance between the light source and the detector was not as farther as better, when the distance was larger than 3.1 cm, the energy density of the emitted photon decreased rapidly, that seriously affected the detected signal quality.51 The PE was simulated in this study by produced different degrees of hypoxemia to simulate different levels of embolism at the pulmonary artery. The results showed that there were significant differences in optical performance of different PE levels in Fig. 6. The drop of light intensity reflected the embolism in the blood. The region beneath coverage of the NIRS probe contributes more sensitivity to NIRS measurements, and scattered photons outside this region can also be detected but with less reliability and sensitivity. This is a common problem with NIRS technology. Furthermore, as mentioned above, the light intensity variations could be a comprehensive outcome of various factors in the detected area, and light intensity as a single contrast agent sometimes may possibly issue a false alarm for embolism. The combination of multivariate modeling such as oxygen consumption and optical properties may make the prediction of PE more reliable. Nevertheless, to the best of our knowledge, this study was the first to study PE using an optical noninvasive approach. From the simulation result, the light intensity could be an indicator of PE determination. In this study, the simulation experiment was carried out under ideal conditions, including the probe contacting the skin surface with zero distance and the probe not causing any pressure to the skin. The thickness and optical properties of the skin did not change. However, in human experiments, the light intensity is influenced by many factors, such as the optical contact distance between probe and tissue, optical properties and thickness of the skin, and the pressure of the probe to the tissue. Based on the simulation results, more real conditions will be considered in the future work of simulation and instrument design. Lightweight detectors might be used for constructing the instrument to reduce the pressure between the probe and the skin and reduce the influence to the detected light intensity. In addition, the tissue optical properties also change with the human exhalation and inhalation. In this study, the average value of the light intensity at exhalation and inhalation status was calculated to eliminate this change influence that represented a relative ideal condition. More complicated reality conditions of exhalation and inhalation will be considered in the future. In conclusion, the present study employed the thoracic model of visible Chinese human and Monte Carlo simulation to investigate noninvasive detected of the PE levels. The study found that photons could reach the pulmonary artery tissue and the light intensity was linearly related to the degrees of embolism. The appearance of PE can produce lower intensity, which could be used as a reflection on the presence of embolus in pulmonary artery. Overall, this study demonstrated the great potential of NIRS in detecting PE. DisclosuresThe authors have no relevant financial interests in this article and no potential conflicts of interest to disclose. AcknowledgmentsThis study was supported by the National Natural Science Foundation of China (Grant No. 81971660), Tianjin Outstanding Youth Fund Project (Grant No. 20JCJQIC00230), Sichuan Science and Technology Program (Grant No. 2021YFH0004), Medical & Health Innovation Project, Chinese Academy of Medical Sciences, & Peking Union Medical College (Grant Nos. 2021-I2M-1-058, 2021-I2M-1-042, 2022-I2M-C&T-A-005, and 2022-I2M-C&T-B-012). ReferencesJ. Gribbin et al.,
“Incidence and mortality of idiopathic pulmonary fibrosis and sarcoidosis in the UK,”
Thorax, 61 980
–985 https://doi.org/10.1136/thx.2006.062836 THORA7 0040-6376
(2006).
Google Scholar
K. A. Martin et al.,
“Time trends in pulmonary embolism mortality rates in the United States,”
J. Am. Heart. Assoc., 9 e016784 https://doi.org/10.1161/JAHA.120.016784
(2020).
Google Scholar
R. W. Yeh et al.,
“Population trends in the incidence and outcomes of acute myocardial infarction,”
N. Eng. J. Med., 362 2155
–2165 https://doi.org/10.1056/NEJMoa0908610 NEJMBH
(2010).
Google Scholar
F. Klok, M. Delcroix and H. J. Bogaard,
“Chronic thromboembolic pulmonary hypertension from the perspective of patients with pulmonary embolism,”
J. Thromb. Haemostasis, 16 1040
–1051 https://doi.org/10.1111/jth.14016
(2018).
Google Scholar
T. Li et al.,
“Simultaneous measurement of deep tissue blood flow and oxygenation using noncontact diffuse correlation spectroscopy flow-oximeter,”
Sci. Rep., 3 1385 https://doi.org/10.1038/srep01358 SRCEC3 2045-2322
(2013).
Google Scholar
T. Li et al.,
“Bedside monitoring of patients with shock using a portable spatially-resolved near-infrared spectroscopy,”
Biomed. Opt. Express., 6 3431
–3436 https://doi.org/10.1364/BOE.6.003431 BOEICL 2156-7085
(2015).
Google Scholar
T. Li, Q. Luo and H. Gong,
“Gender-specific hemodynamics in prefrontal cortex during visual verbal working memory by near-infrared spectroscopy,”
Behav. Brain Res., 209
(1), 148
–153 https://doi.org/10.1016/j.bbr.2010.01.033 BBREDI 0166-4328
(2010).
Google Scholar
B. Pan et al.,
“Noninvasive and sensitive optical assessment of brain death,”
J. Biophotonics, 12 e201800240 https://doi.org/10.1002/jbio.201800240
(2019).
Google Scholar
K. B. Gan, E. Zahedi and M. A. Ali,
“Transabdominal fetal heart rate detection using NIR photoplethysmography: instrumentation and clinical results,”
Trans. Biomed. Eng., 56
(8), 2075
–2082 https://doi.org/10.1109/TBME.2009.2021578
(2009).
Google Scholar
G. Quarto et al.,
“Time-resolved optical spectroscopy of the chest: is it possible to probe the lung?,”
in Eur. Conf. Biomed. Opt.,
(2013). Google Scholar
A. Pifferi et al.,
“Initial non-invasive in vivo sensing of the lung using time domain diffuse optics,”
(2022). Google Scholar
A. W. Dunn et al.,
“In-vitro depth-dependent hyperthermia of human mammary gland adenocarcinoma,”
Mater. Sci. Eng., 69 12
–16 https://doi.org/10.1016/j.msec.2016.06.026 MSCEAA 0025-5416
(2016).
Google Scholar
T. Li et al.,
“Noninvasive diagnosis and therapeutic effect evaluation of deep vein thrombosis in clinics by near-infrared spectroscopy,”
J. Biomed. Opt., 20
(1), 010502 https://doi.org/10.1117/1.JBO.20.1.010502 JBOPFO 1083-3668
(2015).
Google Scholar
S. Yavuz et al.,
“Surgical embolectomy for acute massive pulmonary embolism,”
Int. J. Clin. Exp. Med., 7 5362
–5375
(2014).
Google Scholar
T. Li, H. Gong and Q. Luo,
“Visualization of light propagation in visible Chinese human head for functional near-infrared spectroscopy,”
J. Biomed. Opt., 16
(4), 045001 https://doi.org/10.1117/1.3567085 JBOPFO 1083-3668
(2011).
Google Scholar
T. Li, H. Gong and Q. Luo,
“MCVM: Monte Carlo modeling of photon migration in voxelized media,”
J. Innov. Opt. Health Sci., 3 91
–102 https://doi.org/10.1142/S1793545810000927
(2010).
Google Scholar
T. Li et al.,
“Photon penetration depth in human brain for light stimulation and treatment: A realistic Monte Carlo simulation study,”
J. Innov. Opt. Health. Sci., 10 1743002 https://doi.org/10.1142/S1793545817430027
(2017).
Google Scholar
S. Zhang et al.,
“The Chinese Visible Human (VCH) datasets incorporate technical and imaging advances on earlier digital humans,”
J. Anat., 204 165
–173 https://doi.org/10.1111/j.0021-8782.2004.00274.x JOANAY 0021-8782
(2004).
Google Scholar
L. Wang, S. L. Jacques and L. Zheng,
“MCML—Monte Carlo modeling of light transport in multi-layered tissues,”
Comput. Methods Prog. Biomes., 47 131
–146 https://doi.org/10.1016/0169-2607(95)01640-F
(1995).
Google Scholar
M. A. Kamran, M. Mannann and M. Jeong,
“Differential path-length factor’s effect on the characterization of brain’s hemodynamic response function: a functional near-infrared study,”
Front. Neuroinform., 12 37 https://doi.org/10.3389/fninf.2018.00037
(2018).
Google Scholar
S. L. Jacques, Monte Carlo Modeling of Light Transport in Tissue (Steady State and Time of Flight), 32 109
–144 Springer, Dordrecht
(2010). Google Scholar
C. R. Simpson et al.,
“Near-infrared optical properties of ex vivo human skin and subcutaneous tissues measured using the Monte Carlo inversion technique,”
Phys. Med., 43
(9), 2465 https://doi.org/10.1088/0031-9155/43/9/003
(1998).
Google Scholar
R. Marchesini et al.,
“Optical properties of in vitro epidermis and their possible relationship with optical properties of in vivo skin,”
J. Photochem. Photobiol. B: Biol., 16
(2), 127
–140 https://doi.org/10.1016/1011-1344(92)80004-F JPPBEG 1011-1344
(1992).
Google Scholar
W. A. Bruls and J. C. Leun,
“Forward scattering properties of human epidermal layers,”
Photochem. Photobiol., 40
(2), 231
–242 https://doi.org/10.1111/j.1751-1097.1984.tb04581.x PHCBAP 0031-8655
(1984).
Google Scholar
A. N. Bashkatov et al.,
“Optical properties of human skin, subcutaneous and mucous tissues in the wavelength range from 400 to 2000 nm,”
J. Phys., 38
(15), 2543 https://doi.org/10.1088/0022-3727/38/15/004
(2005).
Google Scholar
A. M. Nilsson, R. Berg and S. Andersson,
“Integrating sphere measurements of tissue optical properties for accurate photodynamic therapy (PDT) dosimetry laser interaction with hard and soft tissue,”
Proc. SPIE, 2323 47
–57 https://doi.org/10.1117/12.199183 PSISDG 0277-786X
(1995).
Google Scholar
J. F. Beek et al.,
“In vitro double-integrating-sphere optical properties of tissues between 630 and 1064 nm,”
Phys. Med., 42
(11), 2255 https://doi.org/10.1088/0031-9155/42/11/017
(1997).
Google Scholar
A. Roggan et al.,
“Optical properties of circulating human blood in the wavelength range 400 to 2500 nm,”
J. Biomed. Opt., 4
(1), 36
–46 https://doi.org/10.1117/1.429919 JBOPFO 1083-3668
(1999).
Google Scholar
M. Friebel et al.,
“Determination of optical properties of human blood in the spectral range 250 to 1100 nm using Monte Carlo simulations with hematocrit-dependent effective scattering phase functions,”
J. Biomed. Opt., 11
(3), 034021 https://doi.org/10.1117/1.2203659 JBOPFO 1083-3668
(2006).
Google Scholar
Y. Liu et al.,
“Monte Carlo and phantom study in the brain edema models,”
J. Innov. Opt. Health. Sci., 10 1650050 https://doi.org/10.1142/S1793545816500504
(2017).
Google Scholar
I. Nishidate et al.,
“In vivo estimation of light scattering and absorption properties of rat brain using a single-reflectance fiber probe during cortical spreading depression,”
J. Biomed. Opt., 20
(2), 027003 https://doi.org/10.1117/1.JBO.20.2.027003 JBOPFO 1083-3668
(2015).
Google Scholar
S. L. Jacques,
“Optical properties of biological tissues: a review,”
Phys. Med. Biol., 58 R37
–R61 https://doi.org/10.1088/0031-9155/58/11/R37
(2013).
Google Scholar
C. Du et al.,
“Quantification of NIRS data of a blood phantom by spectral multicomponent analysis,”
Proc. SPIE, 2626 130
–140 https://doi.org/10.1117/12.228657
(1995).
Google Scholar
S. Erol et al.,
“Is oxygen saturation variable of simplified pulmonary embolism severity index reliable for identification of patients, suitable for outpatient treatment,”
Clin. Respir. J., 12 762
–766 https://doi.org/10.1111/crj.12591
(2018).
Google Scholar
L. Wu, Y. Lin and T. Li,
“Effect of human brain edema on light propagation: a Monte Carlo modeling based on the Visible Chinese Human Dataset,”
IEEE. Photonics J., 9 1
–10 https://doi.org/10.1109/JPHOT.2017.2743048
(2017).
Google Scholar
W. Liu et al.,
“Monte Carlo modeling of photon migration in realistic human thoracic tissues for noninvasive monitoring of cardiac hemodynamics,”
J. Biophotonics, 12 e201900148 https://doi.org/10.1002/jbio.201900148
(2019).
Google Scholar
D. A. Boas and A. M. Dale,
“Simulation study of magnetic resonance imaging-guided cortically constrained diffuse optical tomography of human brain function,”
Appl. Opt., 44 1957
–1968 https://doi.org/10.1364/AO.44.001957 APOPAI 0003-6935
(2005).
Google Scholar
G. Strangman, M. A. Franceschini and D. A. Boas,
“Factors affecting the accuracy of near-infrared spectroscopy concentration calculations for focal changes in oxygenation parameters,”
Neuroimage, 18 865
–879 https://doi.org/10.1016/S1053-8119(03)00021-1 NEIMEF 1053-8119
(2003).
Google Scholar
Y. Fukui, Y. Ajichi and E. Okada,
“Monte Carlo prediction of near-infrared light propagation in realistic adult and neonatal head models,”
Appl. Opt., 42 2881
–2887 https://doi.org/10.1364/AO.42.002881 APOPAI 0003-6935
(2003).
Google Scholar
J. Steinbrink et al.,
“Determining changes in NIR absorption using a layered model of the human head,”
Phys. Med. Biol., 46 879
–896 https://doi.org/10.1088/0031-9155/46/3/320 PHMBA7 0031-9155
(2001).
Google Scholar
D. R. Snelling et al.,
“A calibration-independent laser-induced incandescence technique for soot measurement by detecting absolute light intensity,”
Appl. Opt., 44 6773
–6785 https://doi.org/10.1364/AO.44.006773 APOPAI 0003-6935
(2005).
Google Scholar
F. Martelli et al.,
“There’s plenty of light at the bottom: statistics of photon penetration depth in random media,”
Sci. Rep., 6 27057 https://doi.org/10.1038/srep27057 SRCEC3 2045-2322
(2016).
Google Scholar
G. E. Strangman, Z. Li and Q. Zhang,
“Depth sensitivity and source-detector separations for near infrared spectroscopy based on the Colin27 brain template,”
PLos One, 8 e66319 https://doi.org/10.1371/journal.pone.0066319 POLNCL 1932-6203
(2013).
Google Scholar
M. J. Page et al.,
“Non-invasive imaging and cellular tracking of pulmonary emboli by near-infrared fluorescence and positron-emission tomography,”
Nat. Commun., 6 8448 https://doi.org/10.1038/ncomms9448 NCAOBW 2041-1723
(2015).
Google Scholar
W. Xiong et al.,
“The relationship between tumor markers and pulmonary embolism in lung cancer,”
Oncotargt, 8 41412 https://doi.org/10.18632/oncotarget.17916
(2017).
Google Scholar
T. Li et al.,
“Optimize illumination parameter of low-level laser therapy for hemorrhagic stroke by Monte Carlo simulation on Visible Human dataset,”
IEEE Photonics J, 10
(3), 6100409 https://doi.org/10.1109/JPHOT.2018.2834740
(2018).
Google Scholar
X. Fang et al.,
“Effect of scalp hair follicles on NIRS quantification by Monte Carlo simulation and Visible Chinese Human Dataset,”
IEEE Photonics J., 10
(5), 1
–10 https://doi.org/10.1109/JPHOT.2018.2865427
(2018).
Google Scholar
N. J. Cuper et al.,
“The use of near-infrared light for safe and effective visualization of subsurface blood vessels to facilitate blood withdrawal in children,”
J. Biomed. Eng., 35 433
–440 https://doi.org/10.1016/j.medengphy.2012.06.007 JBIEDR 0141-5425
(2013).
Google Scholar
T. Li et al.,
“A brief review of OPT101 sensor application in near-infrared spectroscopy instrumentation for intensive care unit clinics,”
Sensors, 17
(8), 1701 https://doi.org/10.3390/s17081701 SNSRES 0746-9462
(2017).
Google Scholar
W. L. M. Izzetoglu,
“Investigation of the source-detector separation in near infrared spectroscopy for healthy and clinical applications,”
J. Biophotonics, 12 e201900175 https://doi.org/10.1002/jbio.201900175
(2019).
Google Scholar
D. Li et al.,
“Near-infrared excitation emission and multiphoton-induced fluorescence of carbon dots,”
Adv. Mater., 30 1705913 https://doi.org/10.1002/adma.201705913 ADVMEW 0935-9648
(2018).
Google Scholar
|
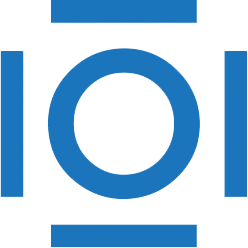
CITATIONS
Cited by 2 scholarly publications.
Tissues
Arteries
Monte Carlo methods
Blood
Near infrared spectroscopy
3D modeling
Tissue optics