|
ACCESS THE FULL ARTICLE
No SPIE Account? Create one
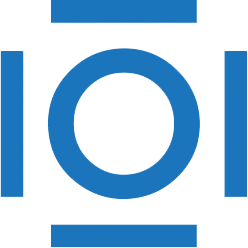
CITATIONS
Cited by 14 scholarly publications and 1 patent.
Bessel functions
Metals
Raster graphics