|
ACCESS THE FULL ARTICLE
No SPIE Account? Create one
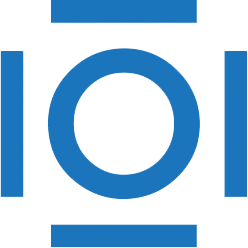
CITATIONS
Cited by 2 scholarly publications.
Neural networks
Critical dimension metrology
Lithography
Cadmium
Data modeling
Photomasks
Error analysis