|
ACCESS THE FULL ARTICLE
No SPIE Account? Create one
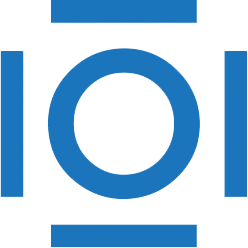
CITATIONS
Cited by 9 scholarly publications.
Reactive ion etching
Neural networks
Etching
Principal component analysis
Data modeling
Gallium
Neurons