Background: E-beam metrologies, both critical dimension scanning electron microscope metrology and defect scan metrology, have been playing a very critical role in gating patterning quality. SEM images can provide rich visual information for engineers to do qualitative and quantitative analyses. However, the low e-beam metrology tool throughput makes it impossible to obtain SEM images for larger area. Monte Carlo-based SEM image simulations or other SEM image simulations require postlithography or postetch pattern three-dimensional structures as prerequisite, and the simulation speed is not sufficiently fast for full chip implementation. Aim: We aim to develop machine learning SEM models with sufficient accuracy and speed for full chip application in semiconductor manufacturing environment. Approach: We have proposed a virtual SEM metrology solution based on U-Net neural network with physics-based feature maps as model input. With information in aerial image space encoded properly, SEM images of both postlithography and postetch can be predicted accurately enough for practical applications using our proposed virtual SEM metrology models. Equipped with GPUs, the machine learning-based SEM image models are fast enough to make it possible to realize full chip SEM generation from post-OPC data. Results: Our machine learning SEM image models can predict SEM images with normalized cross correlation around 0.95 in reference to ground truth SEM images, each SEM image (512 × 512 in size) takes about 800 ms using single CPU, and the speed can be accelerated to about 10 ms with single GPU. Conclusions: Using U-Net structure and physics-based feature maps as model inputs, machine learning-based SEM image models can be developed. The models are sufficiently accurate and fast to find their applications in semiconductor manufacturing, and they can be used as independent model for OPC data verification or generate SEM images as reference for SEM defect scan metrology. |
ACCESS THE FULL ARTICLE
No SPIE Account? Create one
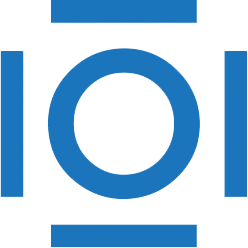
CITATIONS
Cited by 2 scholarly publications.
Scanning electron microscopy
Data modeling
Metrology
Optical proximity correction
Machine learning
3D modeling
Image processing