Purpose: Explainability and fairness are two key factors for the effective and ethical clinical implementation of deep learning-based machine learning models in healthcare settings. However, there has been limited work on investigating how unfair performance manifests in explainable artificial intelligence (XAI) methods, and how XAI can be used to investigate potential reasons for unfairness. Thus, the aim of this work was to analyze the effects of previously established sociodemographic-related confounders on classifier performance and explainability methods. Approach: A convolutional neural network (CNN) was trained to predict biological sex from T1-weighted brain MRI datasets of 4547 9- to 10-year-old adolescents from the Adolescent Brain Cognitive Development study. Performance disparities of the trained CNN between White and Black subjects were analyzed and saliency maps were generated for each subgroup at the intersection of sex and race. Results: The classification model demonstrated a significant difference in the percentage of correctly classified White male (90.3 % ± 1.7 % ) and Black male (81.1 % ± 4.5 % ) children. Conversely, slightly higher performance was found for Black female (89.3 % ± 4.8 % ) compared with White female (86.5 % ± 2.0 % ) children. Saliency maps showed subgroup-specific differences, corresponding to brain regions previously associated with pubertal development. In line with this finding, average pubertal development scores of subjects used in this study were significantly different between Black and White females (p < 0.001) and males (p < 0.001). Conclusions: We demonstrate that a CNN with significantly different sex classification performance between Black and White adolescents can identify different important brain regions when comparing subgroup saliency maps. Importance scores vary substantially between subgroups within brain structures associated with pubertal development, a race-associated confounder for predicting sex. We illustrate that unfair models can produce different XAI results between subgroups and that these results may explain potential reasons for biased performance. |
ACCESS THE FULL ARTICLE
No SPIE Account? Create one
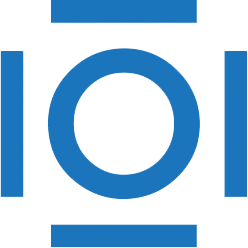
CITATIONS
Cited by 9 scholarly publications.
Brain
Performance modeling
Brain mapping
Cerebellum
Data modeling
Magnetic resonance imaging
Amygdala