Purpose: The quantitative detection, segmentation, and characterization of glomeruli from high-resolution whole slide imaging (WSI) play essential roles in the computer-assisted diagnosis and scientific research in digital renal pathology. Historically, such comprehensive quantification requires extensive programming skills to be able to handle heterogeneous and customized computational tools. To bridge the gap of performing glomerular quantification for non-technical users, we develop the Glo-In-One toolkit to achieve holistic glomerular detection, segmentation, and characterization via a single line of command. Additionally, we release a large-scale collection of 30,000 unlabeled glomerular images to further facilitate the algorithmic development of self-supervised deep learning. Approach: The inputs of the Glo-In-One toolkit are WSIs, while the outputs are (1) WSI-level multi-class circle glomerular detection results (which can be directly manipulated with ImageScope), (2) glomerular image patches with segmentation masks, and (3) different lesion types. In the current version, the fine-grained global glomerulosclerosis (GGS) characterization is provided, including assessed-solidified-GSS (associated with hypertension-related injury), disappearing-GSS (a further end result of the SGGS becoming contiguous with fibrotic interstitium), and obsolescent-GSS (nonspecific GGS increasing with aging) glomeruli. To leverage the performance of the Glo-In-One toolkit, we introduce self-supervised deep learning to glomerular quantification via large-scale web image mining. Results: The GGS fine-grained classification model achieved a decent performance compared with baseline supervised methods while only using 10% of the annotated data. The glomerular detection achieved an average precision of 0.627 with circle representations, while the glomerular segmentation achieved a 0.955 patch-wise Dice dimilarity coefficient. Conclusion: We develop and release an open-source Glo-In-One toolkit, a software with holistic glomerular detection, segmentation, and lesion characterization. This toolkit is user-friendly to non-technical users via a single line of command. The toolbox and the 30,000 web mined glomerular images have been made publicly available at https://github.com/hrlblab/Glo-In-One. |
ACCESS THE FULL ARTICLE
No SPIE Account? Create one
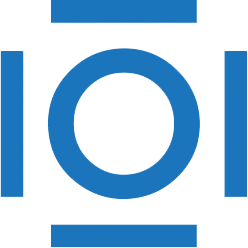
CITATIONS
Cited by 4 scholarly publications.
Image segmentation
Data modeling
Mining
Pathology
Binary data
Image classification
Performance modeling