Purpose: Semi-automatic image segmentation is still a valuable tool in clinical applications since it retains the expert oversights legally required. However, semi-automatic methods for simultaneous multi-class segmentation are difficult to be clinically implemented due to the complexity of underlining algorithms. We purpose an efficient one-vs-rest graph cut approach of which the complexity only grows linearly as the number of classes increases. Approach: Given an image slice, we construct multiple one-vs-rest graphs, each for a tissue class, for inference of a conditional random field (CRF). The one-vs-rest graph cut is to minimize the CRF energy derived from regional and boundary class probabilities estimated from random forests to obtain a one-vs-rest segmentation. The final segmentation is obtained by fusing from those one-vs-rest segmentations based on majority voting. We compare our method to a well-used multi-class graph cut method, alpha-beta swap, and a fully connected CRF (FCCRF) method, in brain tumor segmentation of 20 high-grade tumor cases in 2013 MICCAI dataset. Results: Our method achieved mean Dice score of 0.83 for whole tumor, compared to 0.80 by alpha-beta swap and 0.79 by FCCRF. There was a performance improvement over alpha-beta swap by a factor of five. Conclusions: Our method utilizes the probabilistic-based CRF which can be estimated from any machine learning technique. Comparing to traditional multi-class graph cut, the purposed one-vs-rest approach has complexity that grows only linearly as the number of classes increases, therefore, our method can be applicable for both online semi-automatic and offline automatic segmentation in clinical applications. |
ACCESS THE FULL ARTICLE
No SPIE Account? Create one
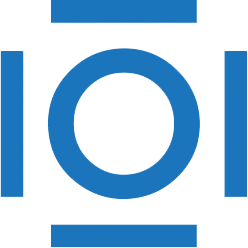
CITATIONS
Cited by 3 scholarly publications.
Image segmentation
Tumors
Medical imaging
Image processing algorithms and systems
Tissues
Brain
Fusion energy