|
ACCESS THE FULL ARTICLE
No SPIE Account? Create one
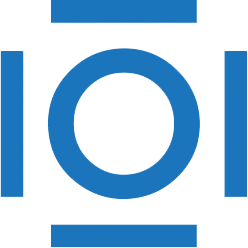
CITATIONS
Cited by 4 scholarly publications and 1 patent.
Image classification
Image processing
Retina
Data modeling
Databases
Convolution
Convolutional neural networks