Rapid and accurate determination of rice diseases is the key to accurate monitoring of agricultural conditions and timely prevention of agricultural yield reduction. Aiming at the problems of low identification accuracy of rice blast and low efficiency of manual detection, we propose an identification method for the six main diseases of rice in the natural environment. At the same time, the identification algorithm of rice false smut is studied. The purpose is to improve the efficiency of the intelligent collection of rice disease images based on convolutional neural network (CNN) and feature matching, so as to identify rice diseases faster. We propose to use SURF and HOG for feature extraction respectively. Then, 20 models were selected using K-nearest neighbors and support vector machine, respectively, for experiments using cross-validation. At the same time, residual network (ResNet) was used to conduct experimental research on six diseases of rice, and the samples were expanded and enhanced. The image scale is increased, and ResNet-20 is used to train the sample data. The experimental model achieved 96.7% accuracy on the test set. The new network achieved 97.4% accuracy. It is nearly 8% points higher than traditional image feature extraction methods. The experimental results show that the improved CNN has high accuracy and a good effect in the identification of main rice diseases. |
ACCESS THE FULL ARTICLE
No SPIE Account? Create one
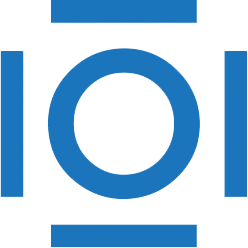
CITATIONS
Cited by 1 scholarly publication.
Convolutional neural networks
Feature extraction
Image segmentation
Image processing
RGB color model
Convolution
Neural networks