The object detectors based on deep learning, such as Yolov3, Yolov3-tiny, and Yolov4-tiny, have achieved real-time object detection in various vision tasks. However, long-tailed distribution of classes, small objects, and incomplete numbers on the dataset have brought challenges to the automatic recognition of wheel-type water meter digit reading using these object detectors. We propose a Mix suppression data augmentation method based on information deletion and resampling. The method improves the long-tailed distribution of the water meter dataset and the performance of small object detection. Moreover, to further improve the performance of detection model, we propose a block spatial attention module to connect with the output of the backbone of object detection model. This module can introduce priori knowledge into the convolutional neural network, reducing the phenomenon of missing objects. The experiments show that our methods successfully improve the performance of neural networks for the water meter digit reading recognition, and they could also be conveniently embedded into other mainstream general object detection models. In addition, we construct a dataset named waterDataset for noncommercial use, which is available at a Github repository: https://github.com/l1158984238/waterDataset. |
ACCESS THE FULL ARTICLE
No SPIE Account? Create one
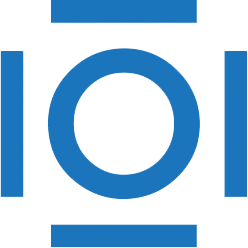
CITATIONS
Cited by 1 scholarly publication.
Bismuth
Head
Performance modeling
Image processing
Data modeling
Convolution
Sensors