Powerful features, which contain more representative information, have become increasingly important in object detection. We exploit the attention mechanism and dilated convolution to strengthen the features used to construct the original feature pyramid network (FPN) and introduce a network that combines the dilated convolution and attention mechanism based on FPN (DAFPN). Specifically, motivated by the attention mechanism, a level-independent attention module (LIAM) is proposed to make high-level feature maps focus on semantic information and low-level feature maps concentrate on spatial information. Meanwhile, we present a pyramidal dilated convolution module (PDCM) that replaces standard convolution with dilated convolution. Instead of previous works that use the same dilation rate for all scales of feature maps, the PDCM applies dilation convolution with various dilation rates to enlarge the effective receptive field of each level’s feature maps suitably. Extensive experiments show that our DAFPN achieves extraordinary performance compared to the state-of-the-art FPN-based detectors on MS COCO benchmark. |
ACCESS THE FULL ARTICLE
No SPIE Account? Create one
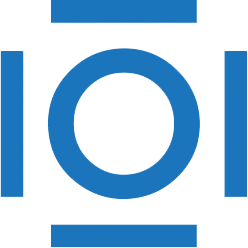
CITATIONS
Cited by 2 scholarly publications.
Convolution
Sensors
Network architectures
Machine vision
Computer vision technology
Convolutional neural networks
Data hiding