Personnel detection in a coal mine is of great significance for monitoring the safety and real-time positioning of underground personnel. However, the uneven illumination, large dust, imaging noise, and other factors give the existing methods a high false detection rate for personnel detection. To overcome this problem, an improved YOLOv4 network for personnel detection based on thermal infrared images is proposed. First, the infrared personnel images are preprocessed by the method of super-resolution convolutional neural network to enhance their resolutions. Second, to improve the detection performance of the YOLOV4 network, the K-means++ clustering is used to optimize the anchor boxes estimation before the network training. Third, to further strengthen the generalization ability of the YOLOv4 and reduce the complexity of network training, the strategy of transfer learning is applied to train the YOLOv4 network. Experimental results show that on the infrared personnel image dataset underground coal mine, the improved YOLOv4 model achieves 96.25% and 48.2 fps in global AP and detection speed, respectively. Moreover, the improved YOLOv4 model has the advantages of strong robustness and generalization ability, which is very suitable for underground personnel detection, and provides a strong guarantee for the safety monitoring and management of coal mine personnel. |
ACCESS THE FULL ARTICLE
No SPIE Account? Create one
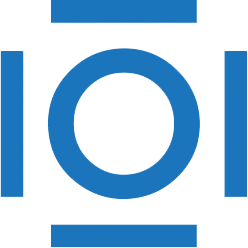
CITATIONS
Cited by 6 scholarly publications.
Infrared imaging
Infrared radiation
Infrared detectors
Land mines
Thermal modeling
Data modeling
Thermography