Face anti-spoofing (FAS) is crucial for safe and reliable biometric systems. In recent years, deep neural networks have been proven to be very effective for FAS as compared with classical approaches. However, deep learning-based FAS methods are data-driven and use learning-based features only. It is a legitimate question to ask whether hand-crafted features can provide any complementary information to a deep learning-based FAS method. To answer this question, we propose a two-stream network that consists of a convolutional network and a local difference network. To be specific, we first build a texture extraction convolutional block to calculate the gradient magnitude at each pixel of an input image. Our experiments demonstrate that additional liveness cues can be captured by the proposed method. Second, we design an attention fusion module to combine the features obtained from the RGB domain and gradient magnitude domain, aiming for discriminative information mining and information redundancy elimination. Finally, we advocate a simple binary facial mask supervision strategy for further performance boost. The proposed network has only 2.79M parameters and the inference speed is up to 118 frames per second, which makes it very convenient for real-time FAS systems. The experimental results obtained on several well-known benchmarking datasets demonstrate the merits and superiority of the proposed method over the state-of-the-art approaches. |
ACCESS THE FULL ARTICLE
No SPIE Account? Create one
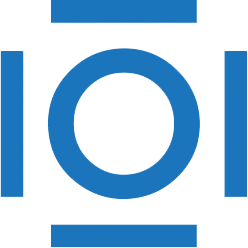
CITATIONS
Cited by 1 scholarly publication.
Binary data
Atomic force microscopy
RGB color model
Video
Feature extraction
Convolution
Data modeling