Deep convolutional neural networks (CNNs) have achieved considerable success in terms of image denoising. However, previous CNN denoisers have been restricted by rigid kernel convolution that applies equal spatial treatment across images. To fully utilize the local differences, we propose a kernel prediction network that examines each pixel region and predicts unique pixel-wise kernels. Several optimizations have been further designed to gather sufficient information for single-image denoising task. We adopt dilated residual blocks to view the local pixel region at varying receptive fields. Then, kernel fusion assembles the information from different scopes and generates accurate kernels for each pixel. Instead of applying the predicted kernels to the original image, we construct a compressed feature map as a substitution such that more relevant local features are collected. Experiments are used to demonstrate that our network achieves favorable results compared with state-of-the-art methods and is adequate for practical applications. |
ACCESS THE FULL ARTICLE
No SPIE Account? Create one
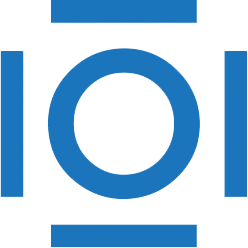
CITATIONS
Cited by 1 scholarly publication.
Denoising
Convolution
Image fusion
Image compression
Feature extraction
Image filtering
Performance modeling