Self-supervised learning of depth from monocular videos has recently drawn attention as it has notable advantages over supervised ones in a training framework. We propose a self-supervised monocular depth estimation method with a set of improvements, which together result in both quantitatively and qualitatively improved depth maps compared to competing self-supervised methods. Our architecture amends current deep convolutional neural network backbone combined with attention mechanism to boost depth estimation performance. Additionally, for addressing moving objects and occlusion, we propose a learnable outlier-masking technique to exclude invalid pixels in photometric error map. Extensive experiments show the effectiveness of the proposed improvements. Our proposed model achieves state-of-the-art performance on KITTI dataset compared with other competing methods. |
ACCESS THE FULL ARTICLE
No SPIE Account? Create one
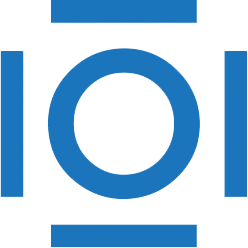
CITATIONS
Cited by 3 scholarly publications.
Cameras
Error analysis
Computer vision technology
Data modeling
Feature extraction
Machine vision
Signal attenuation