1.IntroductionIn this study, we carry out a pilot clinical evaluation of optical technologies for intraoral imaging and photodynamic therapy (PDT). The optical system for image-guided treatment of oral cancers described here is specifically engineered to address challenges faced in resource-limited clinics and is directly motivated by the public health crisis of oral squamous cell carcinoma (OSCC) in South Asia, where widespread chewing of tobacco-based products (e.g., “gutka”) drives truly alarming rates of oral cancer.1 In particular, in India, where there is a lack of widespread access to medical infrastructure for cancer management, the rate of new age-adjusted oral cancer cases is 36% (135,929), and the mortality rate is 42.4% (75,290 deaths). The World Health Organization projected that new oral cancer cases would increase to 209,000 (65%) and death would be 116,000 (65%) in India by 2040.2 Due to a lack of routine oral examination and timely biopsy, late-stage diagnosis is the norm in India, with 70% of cases presenting at stage III-IV.3 As a result, even those patients in rural areas who undergo conventional treatment with surgery and/or chemotherapy show a 5-year survival rate of .4 Clearly, there is an urgent need for new technologies that can help enable timely diagnosis and treatment of oral malignancy in challenging, resource-limited rural clinics. Such technologies must be specifically engineered to be produced at low cost, portable, easy to use, and functional in settings with limited infrastructure. In the present report, we demonstrate the integration of previously reported low-cost technology for PDT with an intraoral optical imaging probe previously validated for oral cancer detection and adapted here for image guidance of PDT.5–7 This work builds upon previous development of a low-cost, LED-based intraoral PDT light delivery system, which was found to be safe and effective in treatment of patients with early oral cancer in India.8 Briefly, patients were photosensitized by systemic administration of aminolevulinic acid (ALA), leading to accumulation of protoporphyrin IX (PpIX). In addition to serving as a photosensitizer for PDT, PpIX also acts as a fluorescence (FL) contrast agent for tumor imaging and treatment guidance.9 We previously reported how a simple smartphone-attached device, consisting of a ring of violet excitation LEDs surrounding an emission filter mounted over the phone camera, could be leveraged as a low-cost solution for imaging PpIX FL in oral lesions.10–12 Although this simple system was leveraged successfully to demarcate lesion positions and estimate the extent of photobleaching after treatment, the physical form factor limited the ability to obtain well-resolved images of lesions inside the oral cavity. Also this use of a single emission further limits the capability to separate PpIX emission from autofluorescence (auto-FL) background. It has been reported previously that analyzing PpIX FL emission relative to tissue auto-FL (red to green channel ratio) improves specificity though the previous single-channel smartphone-based device did not allow for this.13,14 Here we report the adaptation of an oral cancer screening device for use in a new context for intraoral PDT treatment guidance.15–18 This intraoral probe has undergone a pilot phase clinical evaluation in a small cohort of 12 patients (14 lesions) with early stage oral cancer by obtaining multichannel FL and white light images of the lesion before and after PDT treatment.15,16,19 This multichannel FL imaging device allows for ratiometric analysis of PpIX FL emission relative to tissue auto-FL, which has been shown to improve specificity.13,14 We examine the quality of FL image segmentation and the capability to enhance lesion margin visualization during PDT compared to standalone auto-FL, white light imaging, and independently obtained ultrasound (US) images. Bringing together complementary technologies for oral cancer screening and PDT in this manner could be significant as part of a broader effort to deliver therapy at the point of care using low-cost technologies, which can be disseminated to rural clinics.15,16,19 2.Materials and Methods2.1.Subject Selection and Imaging TimelineThe intraoral probe was used for imaging buccal mucosal 14 oral lesions (10 patients each with a unilateral lesion, + 2 patients each with a bilateral lesion, 1 female, 11 males, median age: 39 years, age range: 25 to 53 years). The imaging study was conducted in the Departments of Radiotherapy and Clinical Oncology, Jawaharlal Nehru Medical College, Aligarh Muslim University (AMU), Aligarh, India. The PDT trial was approved by the Indian Council of Medical Research and Dana Farber/Harvard Cancer Center Institutional Review Board (Clinicaltrials.gov, identifier: NCT03638622). All oral lesion sizes were in diameter with histologically proven OSCC (13 lesions with moderately differentiated OSCC, 1 lesion with well-differentiated OSCC). The study subject had been chewing tobacco products (Gutka, Khaini) for many years (7 to 30 years). Patient recruitment was based on inclusion and exclusion criteria. Patients have no history of photosensitivity toward photoactive compounds, including ALA, and no history of malignant disease treatment. Before the FL imaging, the patient was prescribed antibacterial mouthwash (chlorhexidine gluconate 0.2% w/v) for 1 week to decrease the bacterial load at the lesion site and reduce the microbial FL.20,21 White light and auto-FL images were taken before the first oral dose of ALA (DUSA Pharmaceuticals, Inc., Wilmington, Massachusetts, United States), dissolved in fruit juice. The second and third doses of ALA ( each) were given at hourly intervals. Second PpIX FL imaging was taken after the third dose of ALA (Fig. 1). The fractionated schedule of oral ALA administration using three smaller dosesat hourly intervals was chosen to mitigate side effects based on previous literature.22 In a larger patient cohort receiving the same dosage reported previously, one patient experienced hypotension, though it was resolved through hydration.22–24 No post-PDT skin sensitivities were reported though we did observe minor abnormalities in liver and renal function tests after administering ALA, but these quickly resolved without any intervention. Light from an LED source (635 nm peak) was delivered to the lesion surface (total dose at maximum irradiance ) using modular 3D-printed fiber-coupled applicators to facilitate accurate and ergonomic illumination of the target tissue.6,8 The total duration of light delivery was 34 min with two fractions and 2 min intervals in between. The beam spot intensity was uniform to within about 10% variation from center to edge and previous cell culture validation experiments showed that with these dose parameters beam spot size correlates almost perfectly with zone of cell killing.5 The third PpIX FL image was taken after PDT treatment to measure PpIX and auto-FL bleaching (see data S2 in the Supplementary Material). Fig. 1Oral lesion imaging using pWL and FL during ALA-PDT at three timepoints. As shown in this figure, imaging sessions were carried out pre-PDT (immediately prior to ALA administration, for which only background auto-FL is expected), post-ALA (after ALA photosensitization but prior to light delivery, strong PpIX FL signal expected), and post-PDT (after light dose, in which point photobleaching is expected. ![]() 2.2.Intraoral DeviceThe intraoral probe used here was originally developed for oral cancer screening, and it was successfully tested for this application on 5000 patients.15–18 In previous studies, the device used only white light and auto-FL imaging modality for the screening using 405 nm light excitation on the lesion. The auto-FL contrast is enhanced by short-pass filters (425 nm, Asahi Spectra, Tokyo, Japan) and a long-pass filter (470 nm, Asahi Spectra) in front of the UV LED and camera since auto-FL wavelength of regular buccal mucosal tissue is , whereas premalignant and malignant buccal mucosal tissue exhibit auto-FL wavelengths ranging from 635 to 705 nm.25,26 Orthogonal linear polarizers (Edmund Optics, Barrington, New Jersey, United States) are placed in front of the camera module and white LEDs, which results in a polarized white light (pWL) image. This device is the third generation of hardware having a dual-mode, wide field of view for FL imaging and pWL imaging. Device components include a commercial smartphone (Moto G5 Android), a handheld intraoral imaging probe, a light-emitting diode driver, a smartphone case attached to a rechargeable lithium battery, and mobile application software (Fig. 2). Both the back of the smartphone case and attached intraoral probe have four 405 nm Luxeon UV U1 LEDs (Lumileds, Amsterdam, Netherlands) for FL imaging and four 4000-K Luxeon Z ES LEDs for pWL imaging. PpIX has broad Soret band absorption around 410 nm and is simultaneously excited along with lesion site auto-FL. The uniformity of illumination was measured to be 83.80% for pWL and 89.84% for UV light. The probe head has a horizontal aluminum stiffener/heatsink that sits between its two halves, which is securely clamped in place. This helps to keep the probe stable and connected, along with the screw capture notches. To minimize the duration of white light exposure and photobleaching of PpIX and intrinsic auto-FL, both pWL and UV light have a minimum exposure time of 30 ms. To prevent the transmission of oral infections, a sterile sleeve (TIDI Products, Neenah, Wisconsin, United States) was utilized during the imaging of oral lesions. This sleeve was placed over the intraoral probe surface to prevent any contact with the buccal mucosal surface, as demonstrated in Fig. 2. During imaging, the distance between the intraoral probe and the lesion surface was nearly constant (20 to 25 mm), and intensity signals were the same within 20 to 25 mm range. This constant distance is attributed to the probe’s working distance of 20 mm and a narrow depth of field of 5.0 mm, where the smartphone-attached probe gets the sharpest imaging at from the lesion surface. Fig. 2The intraoral imaging probe and attached smartphone. (a) The probe has a semi-flexible intraoral probe head with a camera connected to an MotoG android smartphone via USB. Additionally, another identical camera is installed on the backside of the phone case for whole-mouth imaging. (a), (c) To maintain hygiene during patient testing, the probe is covered with a hygienic sleeve (TIDI Products, Neenah, Wisconsin). (b) The flexible intraoral probe can be adjusted to bend up to 45 deg in either direction, allowing for lesion location examination in anterior, posterior buccal mucosa, and near/at retro-molar trigone. ![]() The long-pass filter in front of the CMOS sensor, blocks the excitation light around 405 nm, enabling it to get the lesion-generated PpIX FL in our PDT study. The existing mobile imaging system has two imaging mode options with camera (Omnivision OV5648 integrated CMOS image sensor): (1) intraoral imaging with the flexible intraoral probe (with a front view of the phone case) and (2) whole mouth imaging with the phone camera (back view of the phone case). This semi-flexible intraoral probe has increased clinical ergonomics. It can be bent to access all areas of the oral cavity where the probe is straight, bent for inward imaging and outward imaging (Fig. 2). The silicone (Mold Star 20Tand Black Silc Pig, Smooth-On, Macungie, Pennsylvania, United States) section of the probe provides flexibility up to 470% elongation before breaking. A thick, 11 mm wide piece of aluminum sheet metal is embedded in the flexible section to maintain the probe head angle after bending to the desired position. It also serves as a thermal sink for the illumination LEDs. Each generated RGB image has the property of dimension and 8-bit depth. Due to its simplicity in imaging, this device could be easily used by a non-specialist in rural areas equipped with low-resource clinical settings. 2.3.In Vitro, Intraoral Device-Based Protoporphyrin IX Fluorescence ImagingThe device was calibrated on the head, and neck cancer OSCC cell line TR146 (cat. no. ECACC 10032305, source histology: well-differentiated keratinizing squamous cell carcinoma), which were used for initial in vitro imaging studies. Here TR146 cells were cultured in two T-flasks at 37°C in Ham’s F-12K (Kaighn’s) medium containing L-glutamine and sodium bicarbonate buffer system and supplemented with a 10% FBS, penicillin/streptomycin, amphotericin-B. The cells were trypsinized (trypsin 25%) and harvested when cell density was achieved around in each T-flask. Cells were incubated with 3 mM ALA (5-ALA HCI, Sigma Aldrich, Israel) for 4 h in a new T-flask. ALA incubated cells were pellet down after 5 min centrifugation at 5000 rpm. TR146 generated PpIX FL images were taken in disposable polymethyl methacrylate (PMMA or “acrylic”) cuvettes, where aggregated cells (dissolved in ) were pipetted down in 2% (w/v) sodium alginate (SA) hydrogel phantom (crosslinked by DPBS supplemented with and ions). PpIX FL intensities were calibrated at various concentrations of PpIX (0 to ) in () tissue phantom.7,27,28 2.4.Lesion Image SegmentationImages were saved as an 8-bit unsigned integer RGB image and split into red and green channels (using Python NumPy module), where dominantly green channel represents the auto-FL, and red channel represents PpIX FL.13,14 A lesion with margins and non-malignant surrounding tissue was segmented on relative red (PpIX) and green (auto-) FL intensity (-value, range: 0 to 4). The factor of 4 was arbitrary to expand the -value scale over a larger range. The red () and green () pixel intensity were normalized on total RGB intensity at the position of th pixel ( ) [Eqs. (1)–(3)]. The Python OpenCV package was used for FL and -value image segmentation29 (see image processing Python codes and methods in the Supplementary Material): 2.5.Statistical AnalysisThe central value of each lesion site and in vitro parameters (i.e., red, green FL parameters, correlation of phantom produced PpIX FL intensity-concentration) were analyzed using R (Comprehensive R Archive Network).30 The difference between mean values of red and green intensities and their relative values during three-time points, pre-ALA, post-ALA, and post-light, was assessed using the Kruskal Wallis (KW) test, an alternate one-way ANOVA test. The Cleveland dot plot was drawn to reduce the clutters of the bar graph using the ggplot2-based “ggpubr” package in the R console. The significance values are considered by calculating the -values at: ***, **, and *. 3.Results and Discussion3.1.Device Performance Using Tissue PhantomsWe initially assessed the intraoral device in the laboratory using PpIX phantoms ( and SA) and a cell culture-based tissue model (ALA photosensitized TR146 cultures). The TR146 cell line was used as a tissue phantom model since it has been well characterized and previously applied for constructing an in vitro human buccal epithelium model for buccal drug targeting.31 Here we also used as a well-established tissue phantom.27 Liquid is a scattering agent and recapitulates a similar wavelength-dependent scattering coefficient as in oral malignant tumor tissue (: ).32 We took images just after stirring the solution as it has the suspension form in most of the media. The range of concentration PpIX (0 to ) in () was based on physiologically generated PpIX after ALA administration in the human body.28 At a lower concentration of PpIX (), the intraoral probe detects the red FL in the cuvette [Figs. 3(a) and 3(b)]. TR146 aggregated and dissolved in SA phantom showed the generated PpIX FL. The cuvette wall on edge departs the green auto-FL and is differentiated with contrast in FL signal from non-ALA treated controls having only untreated TR146 in alginate hydrogel medium. The PpIX FL intensity signal increases linearly with increasing PpIX concentration. The regression correlation shows the robust coefficient of determinant () value within a 95% confidence interval (gray confidence band on the plot) [Figs. 3(c) and 3(d)].33,34 3.2.Clinical Evaluation of Intraoral Probe by PpIX, Autofluorescence, and Ratiometric ImagesIn general, it is difficult to differentiate cancerous from healthy buccal mucosa by traditional clinical oral evaluation (COE) with white light. The reported sensitivity of COE is 93%, though specificity is only 31% due to a high number of false positives.35 To improve specificity, we combined lesion-specific PpIX (90% sensitivity and 60% specificity)36 and auto-FL (variation in reported 30% to 100% sensitivity, 6% to 100% specificity)37 to increase specificity and sensitivity as compared to either alone signal.13,14,38,39 This feature, combined with the tendency of PpIX to accumulate in malignant tissue leads to an elevated ratio of red to green FL emission in malignant versus normal tissues. As such, ratiometric measurement of both PpIX and auto-FL has been shown to improve the accuracy of distinguishing malignant lesions.13,14 Moreover, the ratio has been shown to correlate with the progression of premalignant lesions from mild to severe dysplasia.13,14,40,41 With our RGB multichannel device the green channel is predominantly auto-FL, the red channel represents PpIX FL (Fig. 4). The auto-FL attributed to normal tissue’s intrinsic fluorophores, such as collagen, flavin adenine dinucleotide (FAD), nicotinamide adenine dinucleotide (NADH), and elastin. The combination of these fluorophores produces a broad FL spectrum after the near UV excitation (300 to 450 nm).42 Several clinical and non-clinical studies demonstrated the decreased auto-FL intensity in the tumor tissues compared to normal tissues. In particular, collagen and elastin contain aromatic amino acids that give them strong FL linked to how cells and tissue are structured as connective tissue under the epithelium. The progression of mucosa to premalignant and malignant stages involves a gradual breakdown of the collagen crosslinking associated with the carcinogenesis. This breakdown leads to a decrease in collagen FL. Additionally, mitochondrial fluorophores (NADH and FAD) and endogenous porphyrin are related to metabolic processes in mucosal epithelial tissue.43–45 Studies have shown that as epithelial cells progress from premalignant to malignant, their tissue metabolic activity increases. This leads to a rise in NADH FL and a decrease in the redox ratio (FAD/NADH + FAD).46–48 Fig. 4Image segmentation of FL and white light images (pre-ALA, post-ALA, and post-PDT). The red and green channels correspond largely to PpIX FL and auto-FL, respectively. ![]() In this study, we utilized an excitation wavelength of 405 nm to detect a combination of NADH and FAD signals, which produced an FL peak ranging from 490 to 520 nm.49 However, according to the study, 405 nm excitation did not produce the collagen signal to predict the tissue structure, as stromal collagen predominantly absorbs light at around .49 Using the 405 nm excitation, we avoided confusing keratin FL signals, which can often resemble collagen signals.49,50 This is important because keratinization-related lesions can produce false collagen signals in premalignant and malignant tissues.51,52 This auto-FL property has been exploited in demarcating a lesion with malignant, non-malignant, oral dysplasia (mild to severe dysplasia).18,53,54 It is important to note that the epithelial auto-FL (of FAD, NADH) and PpIX FL observed are measured only on the tissue surface as 405 nm excitation light used here reaches a depth of only about 1 mm in mucosal tissue.55 After ALA administration, the red channel shows strong PpIX FL (Fig. 4). The bleaching of PpIX FL after PDT visually confirms the area of light delivery, including surrounding margins. In contrast, auto-FL decreases after ALA administration, but intrinsic fluorophores also bleached after light delivery (see data S1 in the Supplementary Material). In interpreting the photobleaching data, it is important to again recognize that the blue/violet excitation used here has limited penetration and as such is sampling a small volume relative to the treated volume using red light with penetration of several millimeters. For example, Dimofte et al.56 noted that light delivery for ALA PDT treatment of similar head and neck lesions using a nearly identical excitation wavelength around 635 nm achieves penetration of about 3.4 mm. As a result, the assessment of photobleaching is highly biased toward the tissue surface in the measurements reported. However, as noted above, the use of 405 nm light in this study also played a critical role in excitation of auto-FL, which is used to enhance selectivity of tumor imaging. It is also worth noting that the hardware used here does not allow for measurement of tissue optical properties, which have been shown by others to change during PDT in similar lesions.57,58 Further, based on relative FL pixel intensity (denoted as -value), the image segmentation shows excellent demarcation of lesion FL (Figs. 5 and 6). The increased -value corresponds to a relative increase in PpIX FL and a reduction of auto-FL intensity [, Eq. (3)]. In the earlier studies, the relative FL intensity of split channels (R, G, B) predicts the malignant and normal tissue.40,41 Sharwani et al.14 differentiated not only malignant tissue but also oral premalignant lesion stages, such as dysplasia, hyperplastic tissue, and inflammation, where dysplastic tissue and carcinoma in situ (CIS) showed a higher -value with 83% to 90% sensitivity and 79% to 89% specificity. The Sharwani et al.’s study set the threshold value of -value at 1.2 to differentiate the normal to dysplastic/CIS tissue, but in our image segmentation, threshold value bar (-value) was set at 1.4, which also includes the normal margin (non-malignant/dysplastic) in addition to the lesion (Fig. 6). This low-threshold value segmentation guides the PDT light applicator on the lesion surface with a margin for targeted light dosimetry (see data S2 in the Supplementary Material: 14 lesion’s -value images and -value range: 1.65 to 3.11). Fig. 5Ratiometric analysis of multichannel FL images acquired before photosensitization (Pre-ALA), after photosensitization (post-ALA), and after therapeutic light delivery (post-PDT). Red channels show the lesion site PpIX FL and post-PDT PpIX bleaching. The auto-FL signal in the green channel significantly decreases in both post-ALA as well as in post-PDT as compared to pre-PDT. Ratiometric -value (in the range of 0 to 4) explicitly shows the PpIX FL with clear margins. Further, pseudo-colormesh -values with steps = 0.3 was used for image segmentation and lesion site -value quantification. ![]() Fig. 6Segmentation of images acquired using the intraoral probe after ALA photosensitization. FL image (a) is split into red (PpIX FL) and green (auto-FL) channel images with pseudo-color 16LUT, (b), (c) inset. (d) The relative -value visualized in the Python color map (cmap) displays the pseudo “jet” colors corresponding to -values. (e), (f) The -value thresholding at 1.4 and higher-end 1.8 show the masking of lesions with 10 to 9 mm diameter size. The red-colored masked regions show corresponding mean -values. ![]() The post-ALA images showed a significant decrease in auto-FL. This intrinsic FL (auto-FL) tried to recover as pre-ALA with little gain where the -value difference ( [Pre-ALA − Post-PDT]) is non-significant (Fig. 7). The post-ALA -value of the cancerous lesions was 2.23 (mean) as compared to normal tissues (mean = 0.68), which indicates the cancer lesion specific PpIX synthesis and less auto-FL. This difference between -value among pre-ALA and post-ALA is very significant () as compared to the normal buccal mucosa, where -value at three-time points (pre-PDT, post-ALA, and post-PDT) are almost identical with no significant difference (range = 0.29 to 0.33). The photobleaching manifested by a reduction of the -value from post-ALA to post-PDT by 3.2 times. The change in red-channel FL intensity confirmed the total bleaching of PpIX by 3.1 times (). 3.3.Comparison of Fluorescence with UltrasoundIn order for PDT to achieve a complete tumor response, it is essential that light delivery fully covers the lesion surface with healthy margins. In our clinical study, PDT light delivery used a 3D-printed intraoral applicator-prop applied on the lesion surface. The red LED light from the applicator produces a 20-mm red spot encompassing the lesion (lesion size; , stage) (Fig. 8). PDT light covers the lesion with healthy margins and delivers the calculated light dose. The lesion size parameters were also established by prior US imaging, where the maximum width of 14 lesions is . Here we compare the FL lesion image-based margin parameter with US as it is reported as a gold standard for primary tumor detection with sensitivity and specificity ranging from to 100%.59 The maximum lesion width parameter is quantified using pWL, auto-FL, PpIX FL, and -value segmentation. The boxplot of auto-FL showed a wide range distribution of lesion width [interquartile range (IQR), 6.5] [Fig. 8(d)] in contrast to the -value segmentation distribution (IQR, 3.0). However, the difference in the mean values was insignificant among these imaging methods due to a limited number of lesions. Hence, we analyzed each lesion’s width parameter pairwise with the US using the Cleveland dot plot. The width difference between US with other imaging methods was considered significant (marked red rectangle; Fig. 9) if the difference between pairs is . Only two lesions’ widths in -value segmentation showed a significant difference. The width of -value segmented lesions is nearly identical in most US--value pairs [Fig. 9(d)]. Fig. 8(a)–(c) Comparison of lesion size measured by pWL, PpIX FL, and US. The checkered reference scale predicts the lesion diameter, which is covered by PDT light source beam spot (20 mm.) on lesion. Here PDT light delivered by 3D-printed intraoral applicator. (d) Box plot of distribution of lesion width among 13 lesions with IQR. ![]() Fig. 9(a)–(d) Cleveland dot plot comparisons of the maximum width of lesion measured by pWL, PpIX FL, and US. The dot plots show lesion width among four pairs of pWL, US, auto-FL, PpIX, and segmented -value. The marked red box represents the significant difference in lesion width parameter measurement (i.e., .). ![]() 4.ConclusionIn this study, a smartphone-coupled handheld intraoral probe was used to image PpIX FL before and after PDT in a small cohort of patients who were undergoing PDT treatment for early stage oral cancer. In post-PDT images, the PpIX bleached area confirmed the area of therapeutic () light delivery. The histopathological findings from PDT-treated tissues confirmed that the necrosis had reached a depth of 5 mm, which is reasonably consistent with expectations for light penetration at this wavelength.23,60,61 The 14 treated lesions showed a post-ALA -value range of 1.65 to 3.11, whereas only one lesion with a low -value of 1.75 showed post-PDT recurrence during follow-up (see Table S1 in the Supplementary Material). This patient with incomplete tumor response had moderately differentiated squamous cell carcinoma (mod. diff. SCC) compared to successful ones with well-differentiated squamous cell carcinoma (well. diff. SCC). The successfully treated patients showed no residual diseases during 50 weeks of median follow-up and among 14 lesions 13 showed the post-PDT complete tumor response. However, it is important to note this was a pilot phase evaluation of imaging hardware being used in a new clinical context and image data will need to be gathered from a much larger patient cohort before conclusions about correlation with imaging and later outcomes can be substantiated. Future implementation of this hardware should also incorporate measurement of tissue optical properties, which are expected to change during PDT and could account for differences in fluorophore absorption, which are otherwise conflated with changes in subsequent FL emission. While this could be important to more robust interpretation of tissue changes during auto-FL, the overall agreement in lesion segmentation with US indicates that this hardware works well for its primary intended purpose as a handheld tool for helping the clinician demarcate lesion size and position prior to PDT light delivery. Overall the smartphone-coupled intraoral device successfully integrated features of existing auto-FL imaging devices62–66 while achieving the intended suitability for remote and rural healthcare settings. (1) It is portable, lightweight, durable, sustainable, and affordable. (2) It runs on battery power making it suitable for locations with limited infrastructure. (3) The device is connected to a cloud-based web application that automatically links up with the local network, making remote diagnosis quick and easy. (4) The device is user-friendly and can be operated by local community workers with minimal training. (5) The previously reported capabilities for remote diagnosis and mobile deep learning lesion classification can enable a system for providing health workers with triage instructions to guide them. The potential for acceptance of this technology overall as a low-cost, portable, and capable theranostic cancer technology for global health is supported by the wide availability of smartphone in global health settings.67 In the future, large-scale studies will be required to validate and calibrate the -value ratiometric imaging during the monitoring and guidance of PDT. This approach could potentially discriminate between low to severe dysplasia before developing into CIS. It is also worth noting that implementation of this optical approach could be combined with US for assessment of the depth of invasion. For pre-malignant dysplasia, it is reasonable to assume there is no invasion but for invasive tumors, US would play an important role to establish depth of invasion. With appropriate consideration of these factors and if supported by larger scale studies, oral lesions could be screened and treated simultaneously in remote rural clinical settings with the integration of PDT light delivery and intraoral imaging in the same hardware. DisclosuresThe authors have no relevant financial interests in this article and no potential conflicts of interest to disclose. Code, Data, and Materials AvailabilityDetails regarding methods and materials are provided in the Supplementary Material. AcknowledgmentWe would like to gratefully acknowledge the National Institutes of Health, United States, for funding the PDT trial (Grant Nos. UH2 CA1889901 and UH3 CA1889901 to Tayyaba Hasan and Jonathan P. Celli; Grant No. U01 CA279862 to Tayyaba Hasan, Jonathan P. Celli, and Rongguang Liang). We also would like to thank DUSA pharmaceuticals (Sun Pharmaceutical Industries, Mumbai, India) for providing the 5-ALA. We also would like to thank Jawaharlal Nehru Medical College, Aligarh Muslim University (AMU), Aligarh, India, and the clinical investigation team for assistance and productive conversations: Prof. Ibne Ahmed and Dr. Sayema of the Department of Radiodiagnosis, Jawaharlal Nehru Medical College, AMU, Aligarh; and Prof. S. C. Sharma, Dr. Sheikh Abdul Zeeshan, and Dr. Abdur Rahman of the Department of Otorhinolaryngology (E.N.T.), Jawaharlal Nehru Medical College, AMU, Aligarh. We also would like to acknowledge Mayra Teixeira from the College of Science and Mathematics at UMass Boston for assistance in in-vitro data acquisition. ReferencesP. K. Dhillon et al.,
“The burden of cancers and their variations across the states of India: the global burden of disease study 1990-2016,”
Lancet Oncol., 19
(10), 1289
–1306 https://doi.org/10.1016/S1470-2045(18)30447-9 LOANBN 1470-2045
(2018).
Google Scholar
J. Ferlay et al., Global Cancer Observatory: Cancer Tomorrow, International Agency for Research on Cancer, Lyon, France
(2020). Google Scholar
G. Rath et al.,
“Indian council of medical research consensus document for the management of buccal mucosa cancer,”
Indian J. Med. Paediatr. Oncol., 35
(2), 136
–139 https://doi.org/10.4103/0971-5851.138953
(2014).
Google Scholar
S. B. Thavarool et al.,
“Improved survival among oral cancer patients: findings from a retrospective study at a tertiary care cancer centre in Rural Kerala, India,”
World J. Surg. Oncol., 17
(1), 15 https://doi.org/10.1186/s12957-018-1550-z
(2019).
Google Scholar
H. Liu et al.,
“Development and evaluation of a low-cost, portable, LED-based device for PDT treatment of early-stage oral cancer in resource-limited settings,”
Lasers Surg. Med., 51
(4), 345
–351 https://doi.org/10.1002/lsm.23019 LSMEDI 0196-8092
(2019).
Google Scholar
S. Mallidi et al.,
“Platform for ergonomic intraoral photodynamic therapy using low-cost, modular 3D-printed components: design, comfort and clinical evaluation,”
Sci. Rep., 9 15830 https://doi.org/10.1038/s41598-019-51859-6 SRCEC3 2045-2322
(2019).
Google Scholar
J. Hempstead et al.,
“Low-cost photodynamic therapy devices for global health settings: characterization of battery-powered LED performance and smartphone imaging in 3D tumor models,”
Sci. Rep., 5 10093 https://doi.org/10.1038/srep10093 SRCEC3 2045-2322
(2015).
Google Scholar
S. Khan et al.,
“Clinical evaluation of smartphone-based fluorescence imaging for guidance and monitoring of ALA-PDT treatment of early oral cancer,”
J. Biomed. Opt., 25
(6), 063813 https://doi.org/10.1117/1.JBO.25.6.063813 JBOPFO 1083-3668
(2020).
Google Scholar
J. C. Kennedy, R. H. Pottier and D. C. Pross,
“Photodynamic therapy with endogenous protoporphyrin IX: basic principles and present clinical experience,”
J. Photochem. Photobiol. B, 6
(1–2), 143
–148 https://doi.org/10.1016/1011-1344(90)85083-9 JPPBEG 1011-1344
(1990).
Google Scholar
W. E. Grant et al.,
“Photodynamic therapy of oral cancer: photosensitisation with systemic aminolaevulinic acid,”
Lancet, 342
(8864), 147
–148 https://doi.org/10.1016/0140-6736(93)91347-O LANCAO 0140-6736
(1993).
Google Scholar
K. F. Fan et al.,
“Photodynamic therapy using 5-aminolevulinic acid for premalignant and malignant lesions of the oral cavity,”
Cancer, 78
(7), 1374
–1383 https://doi.org/10.1002/(SICI)1097-0142(19961001)78:7<1374::AID-CNCR2>3.0.CO;2-L CANCAR 0008-543X
(1996).
Google Scholar
J. P. Celli et al.,
“Imaging and photodynamic therapy: mechanisms, monitoring and optimization,”
Chem. Rev., 110
(5), 2795 https://doi.org/10.1021/cr900300p CHREAY 0009-2665
(2010).
Google Scholar
M. A. Scott et al.,
“Fluorescence photodiagnostics and photobleaching studies of cancerous lesions using ratio imaging and spectroscopic techniques,”
Lasers Med. Sci., 15
(1), 63
–72 https://doi.org/10.1007/s101030050049
(2000).
Google Scholar
A. Sharwani et al.,
“Fluorescence spectroscopy combined with 5-aminolevulinic acid-induced protoporphyrin IX fluorescence in detecting oral premalignancy,”
J. Photochem. Photobiol. B, 83
(1), 27
–33 https://doi.org/10.1016/j.jphotobiol.2005.11.007 JPPBEG 1011-1344
(2006).
Google Scholar
R. D. Uthoff et al.,
“Small form factor, flexible, dual-modality handheld probe for smartphone-based, point-of-care oral and oropharyngeal cancer screening,”
J. Biomed. Opt., 24
(10), 106003 https://doi.org/10.1117/1.JBO.24.10.106003 JBOPFO 1083-3668
(2019).
Google Scholar
R. D. Uthoff et al.,
“Development of a dual-modality, dual-view smartphone-based imaging system for oral cancer detection,”
Proc. SPIE, 10486 104860V https://doi.org/10.1117/12.2296435 PSISDG 0277-786X
(2018).
Google Scholar
B. Song et al.,
“Automatic classification of dual-modalilty, smartphone-based oral dysplasia and malignancy images using deep learning,”
Biomed. Opt. Express, 9
(11), 5318 https://doi.org/10.1364/BOE.9.005318 BOEICL 2156-7085
(2018).
Google Scholar
B. Song et al.,
“Mobile-based oral cancer classification for point-of-care screening,”
J. Biomed. Opt., 26
(6), 065003 https://doi.org/10.1117/1.JBO.26.6.065003 JBOPFO 1083-3668
(2021).
Google Scholar
S. Khan et al.,
“Clinical assessment of a low-cost, hand-held, smartphone-attached intraoral imaging probe for ALA PDT monitoring and guidance,”
Proc. SPIE, 11940 15
–20 https://doi.org/10.1117/12.2609956 PSISDG 0277-786X
(2022).
Google Scholar
L. O. Hamad et al.,
“Ex vivo photodynamic diagnosis to detect malignant cells in oral brush biopsies,”
Lasers Med. Sci., 25
(2), 293
–301 https://doi.org/10.1007/s10103-009-0712-1
(2010).
Google Scholar
Z. L. S. Brookes et al.,
“Current uses of chlorhexidine for management of oral disease: a narrative review,”
J. Dent., 103 103497 https://doi.org/10.1016/j.jdent.2020.103497
(2020).
Google Scholar
G. D. MacKenzie et al.,
“Optimal conditions for successful ablation of high-grade dysplasia in Barrett’s oesophagus using aminolaevulinic acid photodynamic therapy,”
Lasers Med. Sci., 24
(5), 729
–734 https://doi.org/10.1007/s10103-008-0630-7
(2009).
Google Scholar
S. A. Siddiqui et al.,
“Clinical evaluation of a mobile, low-cost system for fluorescence guided photodynamic therapy of early oral cancer in India,”
Photodiagn. Photodyn. Ther., 38 102843 https://doi.org/10.1016/j.pdpdt.2022.102843
(2022).
Google Scholar
S. Bown et al.,
“ALA PDT for high grade dysplasia in Barrett’s oesophagus: review of a decade’s experience,”
Proc. SPIE, 7380
(13), 203
–211 https://doi.org/10.1117/12.822976 PSISDG 0277-786X
(2009).
Google Scholar
R. J. Mallia et al.,
“Laser-induced autofluorescence spectral ratio reference standard for early discrimination of oral cancer,”
Cancer, 112
(7), 1503
–1512 https://doi.org/10.1002/cncr.23324 CANCAR 0008-543X
(2008).
Google Scholar
D. C. G. De Veld et al.,
“The status of in vivo autofluorescence spectroscopy and imaging for oral oncology,”
Oral Oncol., 41
(2), 117
–131 https://doi.org/10.1016/j.oraloncology.2004.07.007 EJCCER 1368-8375
(2005).
Google Scholar
B. W. Pogue and M. S. Patterson,
“Review of tissue simulating phantoms for optical spectroscopy, imaging and dosimetry,”
J. Biomed. Opt., 11
(4), 041102 https://doi.org/10.1117/1.2335429 JBOPFO 1083-3668
(2006).
Google Scholar
U. Sunar et al.,
“Quantification of PpIX concentration in basal cell carcinoma and squamous cell carcinoma models using spatial frequency domain imaging,”
Biomed. Opt. Express, 4
(4), 531 https://doi.org/10.1364/BOE.4.000531 BOEICL 2156-7085
(2013).
Google Scholar
Team RC,
“R Core Team R: a language and environment for statistical computing,”
(2020). Google Scholar
J. Jacobsen et al.,
“Filter-grown TR146 cells as an in vitro model of human buccal epithelial permeability,”
Eur. J. Oral Sci., 107
(2), 138
–146 https://doi.org/10.1046/j.0909-8836.1999.eos107210.x
(1999).
Google Scholar
P. R. Bargo et al.,
“In vivo determination of optical properties of normal and tumor tissue with white light reflectance and an empirical light transport model during endoscopy,”
J. Biomed. Opt., 10
(3), 034018 https://doi.org/10.1117/1.1921907 JBOPFO 1083-3668
(2005).
Google Scholar
J. Tyrrell, C. Paterson and A. Curnow,
“Regression analysis of protoporphyrin IX measurements obtained during dermatological photodynamic therapy,”
Cancers-Basel, 11
(1), 72 https://doi.org/10.3390/cancers11010072
(2019).
Google Scholar
A. J. Ruiz et al.,
“Smartphone fluorescence imager for quantitative dosimetry of protoporphyrin-IX-based photodynamic therapy in skin,”
J. Biomed. Opt., 25
(6), 063802 https://doi.org/10.1117/1.JBO.25.6.063802 JBOPFO 1083-3668
(2019).
Google Scholar
J. B. Epstein et al.,
“The limitations of the clinical oral examination in detecting dysplastic oral lesions and oral squamous cell carcinoma,”
J. Am. Dent. Assoc., 143
(12), 1332
–1342 https://doi.org/10.14219/jada.archive.2012.0096
(2012).
Google Scholar
A. Leunig et al.,
“Detection of squamous cell carcinoma of the oral cavity by imaging 5-aminolevulinic acid-induced protoporphyrin IX fluorescence,”
Laryngoscope, 110
(1), 78
–83 https://doi.org/10.1097/00005537-200001000-00015
(2000).
Google Scholar
S. Tatehara and K. Satomura,
“Non-invasive diagnostic system based on light for detecting early-stage oral cancer and high-risk precancerous lesions-potential for dentistry,”
Cancers, 12
(11), 1
–15 https://doi.org/10.3390/cancers12113185
(2020).
Google Scholar
N. van der Beek et al.,
“PpIX fluorescence combined with auto-fluorescence is more accurate than PpIX fluorescence alone in fluorescence detection of non-melanoma skin cancer: an intra-patient direct comparison study,”
Lasers Surg. Med., 44
(4), 271
–276 https://doi.org/10.1002/lsm.21134 LSMEDI 0196-8092
(2012).
Google Scholar
E. W. J. van der Breggen et al.,
“Spectroscopic detection of oral and skin tissue transformation in a model for squamous cell carcinoma: autofluorescence versus systemic aminolevulinic acid-induced fluorescence,”
IEEE J. Sel. Top. Quantum Electron., 2
(4), 997
–1007 https://doi.org/10.1109/2944.577329 IJSQEN 1077-260X
(1996).
Google Scholar
W. Zheng, M. Olivo and K. C. Soo,
“The use of digitized endoscopic imaging of 5-ALA-induced PPIX fluorescence to detect and diagnose oral premalignant and malignant lesions in vivo,”
Int. J. Cancer, 110
(2), 295
–300 https://doi.org/10.1002/ijc.20080 IJCNAW 1097-0215
(2004).
Google Scholar
W. Zheng et al.,
“Detection of squamous cell carcinomas and pre-cancerous lesions in the oral cavity by quantification of 5-aminolevulinic acid induced fluorescence endoscopic images,”
Lasers Surg. Med., 31
(3), 151
–157 https://doi.org/10.1002/lsm.10105 LSMEDI 0196-8092
(2002).
Google Scholar
R. Richards-Kortum and E. Sevick-Muraca,
“Quantitative optical spectroscopy for tissue diagnosis,”
Annu. Rev. Phys. Chem., 47 555
–606 https://doi.org/10.1146/annurev.physchem.47.1.555 ARPLAP 0066-426X
(1996).
Google Scholar
R. R. Alfano et al.,
“Light sheds light on cancer—distinguishing malignant tumors from benign tissues and tumors,”
Bull. N. Y. Acad. Med., 67
(2), 143 BNYMAM
(1991).
Google Scholar
S. Andersson-Engels et al.,
“Fluorescence imaging and point measurements of tissue: applications to the demarcation of malignant tumors and atherosclerotic lesions from normal tissue,”
Photochem. Photobiol., 53
(6), 807
–814 https://doi.org/10.1111/j.1751-1097.1991.tb09895.x PHCBAP 0031-8655
(1991).
Google Scholar
N. Vengadesan, P. Aruna and S. Ganesan,
“Characterization of native fluorescence from DMBA-treated hamster cheek pouch buccal mucosa for measuring tissue transformation,”
Br. J. Cancer, 77
(3), 391
–395 https://doi.org/10.1038/bjc.1998.62 BJCAAI 0007-0920
(1998).
Google Scholar
I. Georgakoudi et al.,
“NAD(P)H and collagen as in vivo quantitative fluorescent biomarkers of epithelial precancerous changes,”
Cancer Res., 62
(3), 682
–687
(2002).
Google Scholar
K. Sokolov et al.,
“Realistic three-dimensional epithelial tissue phantoms for biomedical optics,”
J. Biomed. Opt., 7
(1), 148 https://doi.org/10.1117/1.1427052 JBOPFO 1083-3668
(2002).
Google Scholar
A. Pradhan et al.,
“Steady state and time-resolved fluorescence properties of metastatic and non-metastatic malignant cells from different species,”
J. Photochem. Photobiol. B, 31
(3), 101
–112 https://doi.org/10.1016/1011-1344(95)07178-4 JPPBEG 1011-1344
(1995).
Google Scholar
Y. Wu and J. Y. Qu,
“Autofluorescence spectroscopy of epithelial tissues,”
J. Biomed. Opt., 11
(5), 054023 https://doi.org/10.1117/1.2362741 JBOPFO 1083-3668
(2006).
Google Scholar
Y. Wu et al.,
“Depth-resolved fluorescence spectroscopy reveals layered structure of tissue,”
Opt. Express, 12
(14), 3218 https://doi.org/10.1364/OPEX.12.003218 OPEXFF 1094-4087
(2004).
Google Scholar
G. Shklar,
“Patterns of keratinization in oral leukoplakia,”
Arch. Otolaryngol., 87
(4), 400
–404 https://doi.org/10.1001/archotol.1968.00760060402012
(1968).
Google Scholar
C. Rivera and B. Venegas,
“Histological and molecular aspects of oral squamous cell carcinoma (review),”
Oncol. Lett., 8
(1), 7
–11 https://doi.org/10.3892/ol.2014.2103
(2014).
Google Scholar
I. Pavlova et al.,
“Understanding the biological basis of autofluorescence imaging for oral cancer detection: high-resolution fluorescence microscopy in viable tissue,”
Clin. Cancer Res., 14
(8), 2396
–2404 https://doi.org/10.1158/1078-0432.CCR-07-1609
(2008).
Google Scholar
D. Roblyer et al.,
“Objective detection and delineation of oral neoplasia using autofluorescence imaging,”
Cancer Prev. Res. (Phila), 2
(5), 423
–431 https://doi.org/10.1158/1940-6207.CAPR-08-0229
(2009).
Google Scholar
D. Ihara et al.,
“Fluorescence detection of deep intramucosal cancer excited by green light for photodynamic diagnosis using protoporphyrin IX induced by 5-aminolevulinic acid: an ex vivo study,”
J. Biomed. Opt., 25
(6), 063809 https://doi.org/10.1117/1.JBO.25.6.063809 JBOPFO 1083-3668
(2020).
Google Scholar
A. Dimofte et al.,
“Determination of tissue optical properties in PDT treated head & neck patients,”
Proc. SPIE, 8926 89262C https://doi.org/10.1117/12.2037831 PSISDG 0277-786X
(2014).
Google Scholar
T. C. Zhu and J. C. Finlay,
“The role of photodynamic therapy (PDT) physics,”
Med. Phys., 35
(7), 3127
–3136 https://doi.org/10.1118/1.2937440 MPHYA6 0094-2405
(2008).
Google Scholar
S. M. Gallagher-Colombo et al.,
“Measuring the physiologic properties of oral lesions receiving fractionated photodynamic therapy,”
Photochem. Photobiol., 91
(5), 1210
–1218 https://doi.org/10.1111/php.12475 PHCBAP 0031-8655
(2015).
Google Scholar
E. di Martino et al.,
“Diagnosis and staging of head and neck cancer: a comparison of modern imaging modalities (positron emission tomography, computed tomography, color-coded duplex sonography) with panendoscopic and histopathologic findings,”
Arch. Otolaryngol. Head Neck Surg., 126
(12), 1457
–1461 https://doi.org/10.1001/archotol.126.12.1457
(2000).
Google Scholar
A. C. Kübler,
“Photodynamic therapy,”
Med. Laser Appl., 20
(1), 37
–45 https://doi.org/10.1016/j.mla.2005.02.001
(2005).
Google Scholar
H. J. Nyst et al.,
“Is photodynamic therapy a good alternative to surgery and radiotherapy in the treatment of head and neck cancer?,”
Photodiagn. Photodyn. Ther., 6
(1), 3
–11 https://doi.org/10.1016/j.pdpdt.2009.03.002
(2009).
Google Scholar
, “Identafi: oral mucosal visual enhancement system,”
http://www.identafi.net/
(2019).
Google Scholar
H. Sreeshyla et al.,
“VELscope-tissue fluorescence based diagnostic aid in oral precancer and cancer,”
Indian J. Sci. Technol., 6
(1), 41
–46
(2020).
Google Scholar
Y. J. Yan et al.,
“Band-selection of a portal LED-induced autofluorescence multispectral imager to improve oral cancer detection,”
Sensors, 21
(9), 3219 https://doi.org/10.3390/s21093219 SNSRES 0746-9462
(2021).
Google Scholar
S. K. Melanthota et al.,
“Deep learning-based image processing in optical microscopy,”
Biophys. Rev., 14
(2), 463
–481 https://doi.org/10.1007/s12551-022-00949-3 1793-0480
(2022).
Google Scholar
B. Hunt, A. J. Ruiz and B. W. Pogue,
“Smartphone-based imaging systems for medical applications: a critical review,”
J. Biomed. Opt., 26
(4), 040902 https://doi.org/10.1117/1.JBO.26.4.040902 JBOPFO 1083-3668
(2021).
Google Scholar
|
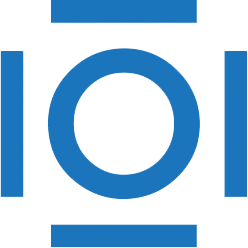
CITATIONS
Cited by 3 scholarly publications.
Photodynamic therapy
Tissues
Image segmentation
Cancer
Biomedical optics
Oncology
Imaging systems