|
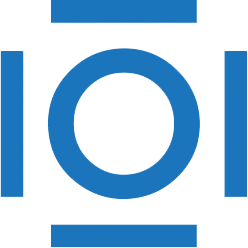
CITATIONS
Cited by 12 scholarly publications.
Aerosols
LIDAR
Atmospheric modeling
Atmospheric particles
Backscatter
Pattern recognition
Databases