Forestry pests pose a significant threat to forest health, making precise extraction of infested trees a vital aspect of forest protection. In recent years, deep learning has achieved substantial success in detecting infestations. However, when applying existing deep learning methods to infested tree detection, challenges arise, such as limited training samples and confusion between forest areas and artificial structures. To address these issues, this work proposes a two-stage hierarchical semi-supervised deep learning approach based on unmanned aerial vehicle visible images to achieve the individual extraction of each pine wilt disease (PWD). The approach can automatically detect the positions and crown extents of each infested tree. The comprehensive framework includes the following key steps: (a) considering the disparities in global image representation between forest areas and artificial structures, a scene classification network named MobileNetV3 is trained to effectively differentiate between forested regions and other artificial structures. (b) Considering the high cost of manually annotating and incomplete labeling of infested tree samples, a semi-supervised infested tree samples mining method is introduced, significantly reducing the workload of sample annotation. Ultimately, this method is integrated into the YOLOv7 object detection network, enabling rapid and reliable detection of infested trees. Experimental results demonstrate that, with a confidence threshold of 0.15 and using the semi-supervised sample mining framework, the number of samples increases from 53,046 to 93,544. Precision evaluation metrics indicate a 5.8% improvement in recall and a 2.6% increase in mean average precision@.5. The final test area prediction achieves an overall accuracy of over 80% and the recall rate of over 90%, indicating the effectiveness of the proposed method in PWD detection. |
ACCESS THE FULL ARTICLE
No SPIE Account? Create one
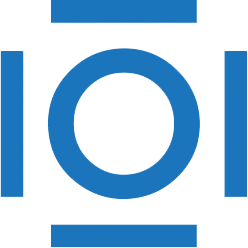
CITATIONS
Cited by 1 scholarly publication.
Object detection
Education and training
Diseases and disorders
Mining
Unmanned aerial vehicles
Scene classification
Deep learning