Most remote sensing sea ice classification methods use single-source remote sensing data, such as synthetic aperture radar (SAR) data and optical remote sensing data. SAR data contain rich sea ice texture information, but the data are relatively single, making it difficult to distinguish detailed sea ice categories. Optical data include abundant spatial-spectral information, but they are often affected by clouds, fog, and severe weather. Hence, given the limitations of single-source data, the remote sensing sea ice classification accuracy cannot be further improved. A sea ice classification method based on deep learning and multisource remote sensing data fusion is proposed utilizing an improved densely connected convolutional neural network (DenseNet) to mine and fuse the multilevel features of sea ice. According to the characteristics of SAR data and optical data, a dual-branch network structure based on an improved DenseNet is employed for feature extraction, and a squeeze-and-excitation attention mechanism is introduced to weight the fusion features to further enhance the feature weights that can effectively distinguish different types of sea ice. The fully connected network is used to perform the deep fusion of features and classify sea ice. To verify the effectiveness of the proposed method, two sets of sea ice data are utilized for sea ice classification. The experimental results show that the proposed method fully excavates and fuses the multilevel characteristics of heterogeneous data using the improved dual-branch network structure, leverages the complementary characteristics of SAR data and optical data, significantly increases the sea ice classification accuracy, and effectively improves the influence of cloud cover on the sea ice classification accuracy. Compared with the typical single-source data classification method and other heterogeneous data fusion classification methods, the proposed method achieves superior overall classification accuracy (98.49% and 98.58%). |
ACCESS THE FULL ARTICLE
No SPIE Account? Create one
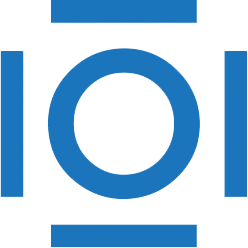
CITATIONS
Cited by 1 scholarly publication.
Ice
Synthetic aperture radar
Data fusion
Remote sensing
Data modeling
Feature extraction
Image fusion