A conventional possibilistic c-mean (PCM)-based algorithm uses means or variance–covariance statistical variables computed from training data sets for classification. Two objectives were covered; in the first objective, the PCM classifier using the training samples as “individual sample as mean” (PCM_IM) in place of “mean” was experimented with handling heterogeneity within the class. The second objective, the PCM-based local convolutional method, was tested with different parameters to handle the noisy pixel. Local convolution algorithms reduce the effect of noise, and PCM_IM handles heterogeneity to enhance the classification performance within the class. The optimized algorithm was first calculated in this research by applying the local convolutional methods for different distance measures and fuzziness factors. This optimized algorithm was obtained by classifying the dense forest, eucalyptus, riverine sand, water, wheat, and grassland classes using the multispectral images of Landsat-8 and Formosat-2 of the Haridwar area. It was observed that the Bray–Curtis distance measures at m = 2.9 (fuzziness factor) for possibilistic local information c-means using the base PCM classifier yields the highest overall accuracy (OA) (86.37%), irrespective of the classifier. Then, PCM_IM provides a classwise and OA that handles heterogeneity within the class. The classwise accuracy of dense forest, eucalyptus, grassland, sand, water, and wheat is 88.12%, 95.29%, 78.79%, 89.17%, 89.72%, and 92.43%, respectively, and the OA is 85.72%, whereas OA of the PCM classifier using the training sample as mean is 75.18%. |
ACCESS THE FULL ARTICLE
No SPIE Account? Create one
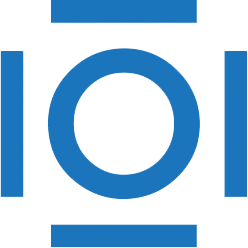
CITATIONS
Cited by 4 scholarly publications.
Distance measurement
Image classification
Earth observing sensors
Fuzzy logic
Landsat
Convolution
Image processing