Hyperspectral image (HSI) classification is major and necessary task related to HSI analysis in the field of remote sensing. The fundamental steps in this task are band selection (BS), spatial feature extraction, and classification. HSIs generally equipped with rich spectral and spatial information and having the properties such as non-stationary and non-Gaussian. To process such rich information, wavelet transform (WT) is the perfect candidate. Also, the multiscale system in wavelets will be used in complete information extraction. So, classification task is implemented by the application of WT in BS and spatial feature extraction. Here, BS is achieved by the combination of clustering based on key band identification and ranking using wavelet entropy (WE). Discrete wavelet transform is applied along three dimensions to extract spatial features. The extracted spectral and spatial features are used in final classification by convolution neural networks classifier. From the obtained results it is observed that, with the advantage of using WT, the proposed method has successfully addressed overfitting and huge data dimensionality problems. Comparison and detailed analysis of the class-wise accuracies also clearly shows the impact of using WT in HSI classification. Evaluation results on three publicly used datasets namely Indian Pines, University of Pavia, and Salinas shows the significant performance over state-of-the-art methods. The proposed method has attained overall accuracy of 93.85%, 99.05%, and 97.13% for the three datasets, respectively. |
ACCESS THE FULL ARTICLE
No SPIE Account? Create one
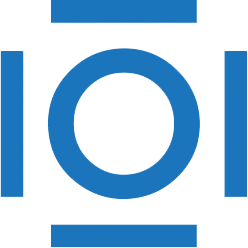
CITATIONS
Cited by 3 scholarly publications.
Wavelets
Feature extraction
Data modeling
Convolution
Hyperspectral imaging
Image classification
3D modeling