The monitoring of threatened habitats is a key objective of European environmental policies. Due to the high cost of current field-based habitat mapping techniques, there is keen interest in proposing solutions that can reduce cost through increased levels of automation. Our study aims to propose a habitat mapping solution that benefits both from the merits of convolutional neural networks (CNNs) for image classification tasks, as well as from the high spatial, spectral, and multitemporal unmanned aerial vehicle image data, which shows great potential for accurate vegetation classification. The proposed CNN-based method uses multitemporal multispectral aerial imagery for the classification of threatened coastal habitats in the Maharees (Ireland) and shows a high level of classification accuracy. |
ACCESS THE FULL ARTICLE
No SPIE Account? Create one
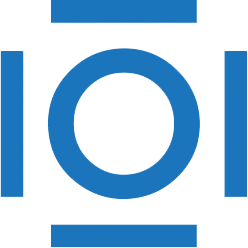
CITATIONS
Cited by 1 scholarly publication.
Animal model studies
Image classification
Associative arrays
Data modeling
Performance modeling
Convolutional neural networks
Coastal modeling