Gross primary productivity (GPP) is a significant indicator of terrestrial system carbon cycle and is one of the most important parameters of global climate change. Accurate modeling of GPP is important for understanding the changes of carbon dioxide in the atmosphere. Although many studies have been conducted to explore methods for estimating GPP, large uncertainties still exist among different models. The combination of remote sensing data with flux data is a feasible approach to determine site-based GPP. Using multisource remote sensing data, meteorological data obtained from Google Earth Engine, and GPP estimates from eddy covariance measurement, we present a model, based on the random forest regression (RFR) algorithm, to predict site-scale GPP. First, we train and tune the RFR model for 16 global deciduous broadleaf forest (DBF) flux sites by remote sensing-based drivers. Then we evaluate the RFR model’s performance by predicting GPP for the other eight DBF sites. Our results show that, compared with the MOD17A2H GPP product, this GPP prediction method improves modeling of GPP, with an R2 of 0.82 and an RMSE of 1.93 g C m − 2 d − 1. Contribution analysis shows that the enhanced vegetation index is the most important driver among input variables. Our study may provide a reliable method to predict GPP in global DBF, which is promising for different-scale evaluations of carbon sequestration. |
ACCESS THE FULL ARTICLE
No SPIE Account? Create one
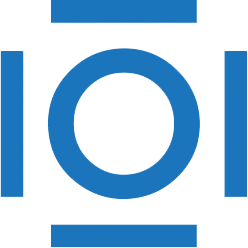
CITATIONS
Cited by 7 scholarly publications.
Data modeling
Remote sensing
Atmospheric modeling
MODIS
Vegetation
Ecosystems
Carbon