|
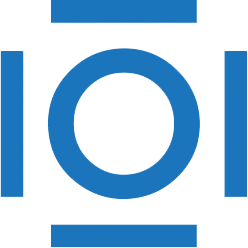
CITATIONS
Cited by 13 scholarly publications.
Vegetation
Associative arrays
LIDAR
Buildings
Data acquisition
Visualization
Image segmentation