|
ACCESS THE FULL ARTICLE
No SPIE Account? Create one
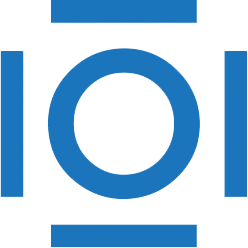
CITATIONS
Cited by 17 scholarly publications.
Convolution
Data modeling
Remote sensing
Classification systems
Visual process modeling
Image classification
Buildings