|
ACCESS THE FULL ARTICLE
No SPIE Account? Create one
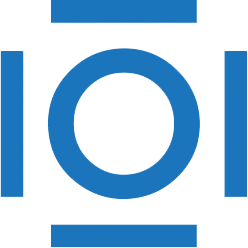
CITATIONS
Cited by 66 scholarly publications.
Neural networks
Remote sensing
Simulation of CCA and DLA aggregates
Image classification
Detection and tracking algorithms
Composites
Image fusion