|
Advances in 3D Human Face Imaging and Automated Facial Expression AnalysisMegan A. Witherow and Khan M. Iftekharuddin Old Dominion University, USA Ubiquitous to interpersonal communication and more universal than language, human facial expressions have been a source of intrigue since the time of Darwin. Widespread applications in psychology, medicine, education, marketing, security, human–computer interactions, and more have motivated decades of research in automated facial imaging and facial expression analysis (FEA). Among many promising methods, FEA using constituent action units (AUs) has gained attention. Advances in modern sensing offer rich 2D and 3D facial imaging data that are amenable to automated FEA. Recent advances in sensing and machine learning (ML), and specifically deep learning (DL) methods, offer advantages in processing 3D imaging data. Thus, sensing and analysis of 3D expressions has emerged as an active multidisciplinary area for optical and digital information processing education and research. Stereophotogrammetry is the use of multiple photographic imaging measurements to estimate the 3D coordinate points of an object, such as a face. Modern 3D stereophotogrammetric imaging systems represent the facial surface with near–ground-truth dense point clouds. These point clouds often undergo preprocessing steps such as 3D image registration and normalization followed by feature extraction from the 3D point cloud data. In a traditional ML pipeline, feature engineering is performed to compute geometric features (such as 3D curvature features) and spatial features (such as geodesic distances between facial landmarks). The features are subsequently input into ML models, such as k-nearest neighbor or support vector machines, for learning and classification. By contrast, DL models learn feature extraction and classification steps directly from the data. Different types of DL models require different input representations for the 3D facial data. Common representations include 3D occupancy grids for models such as 3D convolutional neural networks (CNNs), 3D meshes for graphical CNNs, and raw point clouds for PointNets. Teaching 3D FEA occurs best through hands-on experiments that apply theory to solve real problems. Advances in imaging technology, processing power, and storage capability have opened the doors for experiential learning opportunities in sensing and processing of 3D data. There remain many exciting open research areas for students and researchers. Powerful ML/DL methods have proved promising for robust AU-based analysis of neutral, happy, sad, and surprised expressions. However, expressions of fear and anger, with confounding subtle and co-occurring AUs, remain challenging. Current state-of-the-art 3D imaging and FEA methods still have a long way to go before they are ready to address the challenges of real-world applications such as automated expression analysis in autism intervention. Furthermore, due to large-scale variations in 3D data, existing methods fail to generalize to subjects outside of the training set. Overcoming these exciting challenges will be the next frontier to expanding 3D FEA beyond the laboratory and into real-world applications. |
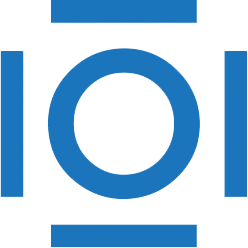
CITATIONS
3D image processing
3D modeling
3D acquisition
Stereoscopy
Finite element methods
3D metrology
3D surface sensing