|
ACCESS THE FULL ARTICLE
No SPIE Account? Create one
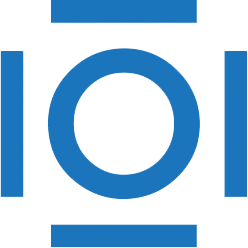
CITATIONS
Cited by 1 scholarly publication.
Remote sensing
Visualization
Image classification
Databases
Sun
Satellites
Classification systems