|
ACCESS THE FULL ARTICLE
No SPIE Account? Create one
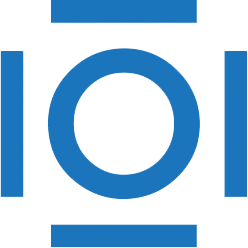
CITATIONS
Cited by 6 scholarly publications.
Stereolithography
Data modeling
Biopsy
Breast cancer
Machine learning
Data mining
Breast