|
ACCESS THE FULL ARTICLE
No SPIE Account? Create one
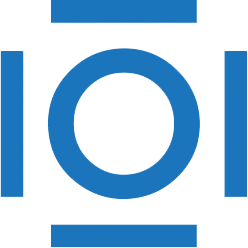
CITATIONS
Cited by 13 scholarly publications and 1 patent.
Data modeling
Magnetic resonance imaging
Reconstruction algorithms
Convolution
Inverse problems
Image restoration
Image filtering