|
ACCESS THE FULL ARTICLE
No SPIE Account? Create one
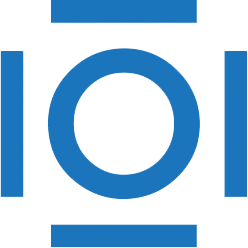
CITATIONS
Cited by 5 scholarly publications.
Image segmentation
Tissues
RGB color model
Image analysis
Cancer
Chemical analysis
Image quality