|
ACCESS THE FULL ARTICLE
No SPIE Account? Create one
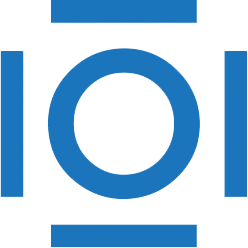
CITATIONS
Cited by 2 scholarly publications.
Image segmentation
Magnetic resonance imaging
Brain
Neuroimaging
Tissues
Pathology
Statistical modeling