|
ACCESS THE FULL ARTICLE
No SPIE Account? Create one
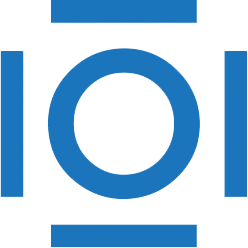
CITATIONS
Cited by 4 scholarly publications.
Reconstruction algorithms
Lawrencium
Image quality
Image processing
Image restoration
Particles
Image classification