|
ACCESS THE FULL ARTICLE
No SPIE Account? Create one
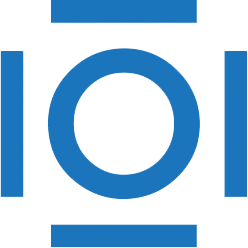
CITATIONS
Cited by 1 scholarly publication.
Lawrencium
Machine learning
Statistical analysis
Probability theory
Error analysis
Algorithms
Matrices