|
ACCESS THE FULL ARTICLE
No SPIE Account? Create one
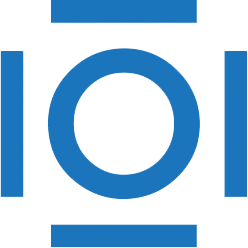
CITATIONS
Cited by 3 scholarly publications.
Water
Error analysis
Hyperspectral imaging
Reflectivity
Optical properties
Remote sensing
Data modeling