|
ACCESS THE FULL ARTICLE
No SPIE Account? Create one
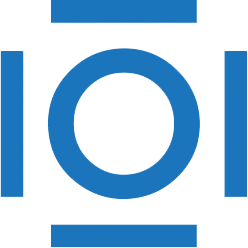
CITATIONS
Cited by 2 scholarly publications.
Image classification
Binary data
RGB color model
Machine learning
Image filtering
Data modeling
Feature extraction