|
ACCESS THE FULL ARTICLE
No SPIE Account? Create one
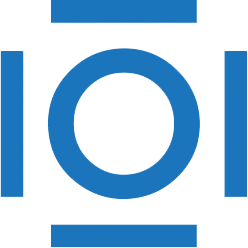
CITATIONS
Cited by 8 scholarly publications.
Roads
Image segmentation
Image classification
Earth observing sensors
Buildings
Satellite imaging
Satellites