|
ACCESS THE FULL ARTICLE
No SPIE Account? Create one
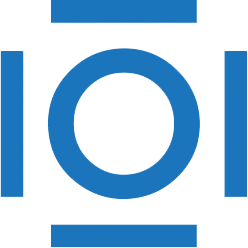
CITATIONS
Cited by 2 scholarly publications.
Autoregressive models
Compressed sensing
Algorithm development
Radon
Signal processing
Linear filtering
Convex optimization