|
ACCESS THE FULL ARTICLE
No SPIE Account? Create one
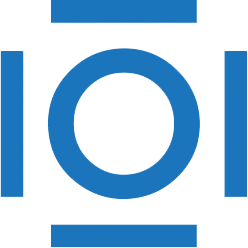
CITATIONS
Cited by 9 scholarly publications and 2 patents.
Associative arrays
Optical character recognition
Detection and tracking algorithms
Image classification
Current controlled current source
Statistical modeling
Data modeling