|
ACCESS THE FULL ARTICLE
No SPIE Account? Create one
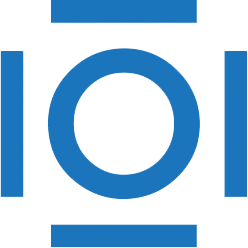
CITATIONS
Cited by 16 scholarly publications.
Nonlinear filtering
Detection and tracking algorithms
Digital filtering
Monte Carlo methods
Particles
Smoothing
Computer simulations