|
ACCESS THE FULL ARTICLE
No SPIE Account? Create one
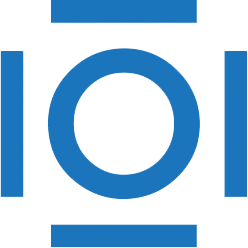
CITATIONS
Cited by 5 scholarly publications.
Expectation maximization algorithms
Detection and tracking algorithms
Error analysis
Monte Carlo methods
Matrices
Statistical analysis
Computer simulations