|
ACCESS THE FULL ARTICLE
No SPIE Account? Create one
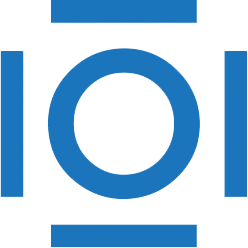
CITATIONS
Cited by 5 scholarly publications.
Diffusion
Super resolution
Image processing
Image restoration
Image analysis
Anisotropic diffusion
Expectation maximization algorithms