|
ACCESS THE FULL ARTICLE
No SPIE Account? Create one
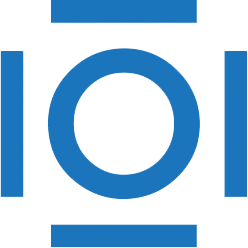
CITATIONS
Cited by 3 scholarly publications.
Target detection
Performance modeling
Image processing
Image segmentation
Automatic target recognition
Image analysis
Sensors