|
ACCESS THE FULL ARTICLE
No SPIE Account? Create one
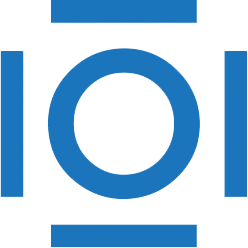
CITATIONS
Cited by 2 scholarly publications.
Quantization
Breast
Magnetic resonance imaging
Diagnostics
Neural networks
Mammography
Computer aided diagnosis and therapy