|
ACCESS THE FULL ARTICLE
No SPIE Account? Create one
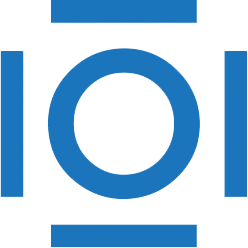
CITATIONS
Cited by 1 scholarly publication.
Neural networks
Data modeling
Thermal modeling
Computer programming
Failure analysis
Humidity
Infrared cameras