|
ACCESS THE FULL ARTICLE
No SPIE Account? Create one
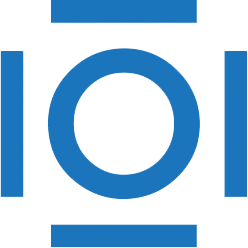
CITATIONS
Cited by 2 scholarly publications.
Visualization
Principal component analysis
Data modeling
Visual analytics
Process control
Dynamical systems
Semiconductors