|
ACCESS THE FULL ARTICLE
No SPIE Account? Create one
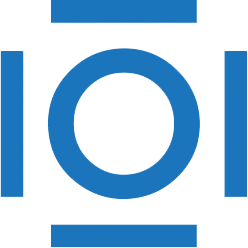
CITATIONS
Cited by 14 scholarly publications.
Target detection
Data modeling
Detection and tracking algorithms
Sensors
Hyperspectral imaging
Hyperspectral target detection
Principal component analysis