|
ACCESS THE FULL ARTICLE
No SPIE Account? Create one
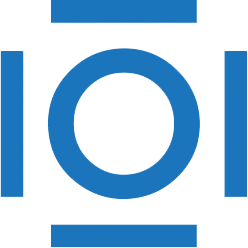
CITATIONS
Cited by 1 scholarly publication and 3 patents.
Data modeling
Neural networks
Autoregressive models
Performance modeling
Temperature metrology
Process modeling
Mathematical modeling